Big data promises fairness. With enough information about individuals and populations, we can design algorithms that will identify the best possible answer to a given question, free of human bias. Algorithms, after all, are not racist, sexist, or elitist.
Or are they?
In her new book, Weapons of Math Destruction, data scientist Cathy O’Neil discusses example after example of algorithms that make troublingly unfair decisions. Algorithms that—under the guise of math, fairness, and objectivity—reinforce and magnify the old biases and power dynamics that we hoped they would eliminate. A former mathematician and finance quant, O’Neil is now turning her technical skills toward the goal of fulfilling that old promise of fairness through big data. (She is arguably the mathiest member of the Occupy Wall Street movement.)
O’Neil and I spoke at Quartz’s offices in New York. We discussed the decisions algorithms are making about us, what’s wrong with them, and her vision for a better algorithmic future. (This transcript has been edited for concision and clarity.)
Quartz: Your book is partially about algorithmic decisions that people aren’t aware of, or that aren’t expected. What are some decisions being made by algorithms that people might not know about?
O’Neil: All of the time when you’re on the internet. All of the time. I have a couple examples that I like to tell because they affect everyone, and everyone is kind of offended by them in a very direct way.
One of them is, you call up customer service, and from your phone number they infer your value as a customer. If you’re a low-value customer you will wait on hold forever. If you’re a high-value customer you get to a customer representative immediately. And if you’re low-value, you’re likely to be told by the rep, “Oh you’re low-value, you’re not going to get what you want.” That happens. I didn’t even know the rep knows your score, but turns out that in that system they actually do. And they can say, “I’m not going to give you what you want.”
QZ: And so most people assume that what’s happening is just some kind of case-by-case human decision?
O’Neil: They figure that when you call, you just get in line. That’s one example. Another example is, you go to Capital One’s website, they infer based on your browsing information what kind of value as a customer you represent, and they’ll show you a different ad based on what I call your e-credit score. Not your actual credit score, because they don’t have access to that based on your profile information. But they kinda make up an ad-hoc credit score at the moment you arrive, and show you high-interest or low-interest advertisements.
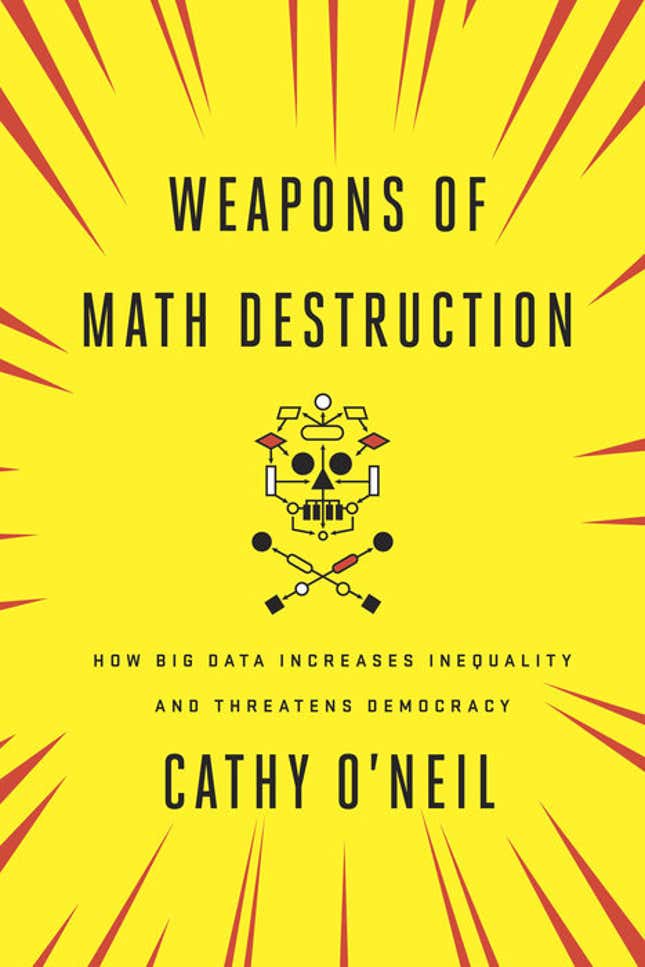
QZ: So to some extent these decisions are made in secret. That’s one of the three characteristics you identify of a dangerous algorithm, what you call a Weapon of Math Destruction, or WMD. Tell us about these characteristics.
O’Neil: The three that I worry about are scale, secrecy, and destructiveness. It’s not just scale alone. The Netflix algorithm is widespread, but I don’t care about it. Maybe because it’s not destructive, but it’s also just not important. The worst that can happen to a person who gets a bad suggestion on Netflix is that they’re like, “That movie sucked.” So I care about scale and importance. It has to affect a lot of people in an important way. That’s why I talk about things like jobs, prison, and insurance.
The second thing is secrecy. People are basically getting or not getting those things that they need based on scores that they don’t understand and sometimes don’t even know exist. Right there you already have something very dangerous. If you have something that’s that important, and it’s secret, that is already something you can object to.
Those first two things are already red flags that should be under scrutiny by society and by regulators. But in addition to that, I feel like I found examples in the book that are also provably destructive. And when I say destructive, it’s not just destructive for the individual, typically—and this is just an observation, really—it’s not just the individual’s life, but it actually engenders a feedback loop that is destructive. A pernicious feedback loop that often undermines its original goal.
QZ: A striking example of a WMD in the book with this kind of feedback loop is the teacher value-added model, which tries to identify bad teachers by comparing their students’ performance from one year to the next. In this case the model seems to have set up various incentives that kind of contradicted each other, leading to a worse outcome overall.
O’Neil: Michelle Rhee in Washington, DC, was this really gung-ho education reformer. She was hired to apply all of these new reform ideas. She instituted both a bonus for people who got good teacher assessment scores, and for principals at those schools, and she would fire teachers with bad enough scores. What happened, we have reason to believe, is that in DC a lot of teachers just cheated. They like, changed the answers on their students’ scores.
It’s obvious to everyone that if you incentivize something like good standardized test scores, then the teachers are going to teach to the test. But it should also be obvious that if there’s enough carrot and/or enough stick, it’s going to be more extreme than that. You’re going to actually see cheating. And that’s what we saw. We saw a dubious and unusual number of erasures at various schools, including one of the schools that was sending kids to a class taught by Sarah Wysocki. So these kids came into her class with very good scores from the previous year—from a school that had unusual numbers of erasures—but who couldn’t read or write at grade level. So it was very suspicious.
And then she got fired based on her teacher assessment score, and she has reason to believe that the expected score for those kids was unreasonably inflated. So she couldn’t meet those expectations. If you have cheating, then the teacher the year after that can’t possibly keep up. And they are going to get dinged for that other teacher’s cheating. Even if that’s not exactly what happened, if you think about it in terms of incentives, this is what’s going to happen. You’re gonna get cheating. It’s just something we should know by now.
QZ: How should we think about what a ”model” or “algorithm” is, generally?
O’Neil: For me, what I mean by this is something that takes in data, and a definition of success, and trains to learn to seek patterns. And then the model becomes a way of predicting. The most important aspect of this thing is not at the technical level; the most important thing is the usage. How is it being marketed? How is it being applied? How are the powers that own it deploying it? The examples I care about are using a model as a sorting mechanism—a scoring system, where people are scored, ranked, and sorted by that score. And then there are serious consequences for a high or a low score.
QZ: How do you think we got to this point where it’s a black box that everyone agrees comes up with a “correct” answer, and that there’s no reason to challenge it?
O’Neil: We have an infrastructure, probably a well-deserved infrastructure in this country, whereby we trust science. Science has done a lot for us. It predicts things like eclipses really well. The sleight of hand that’s happened in the big data era is that we think we can manifestly move that technology onto the human sphere. And we can’t. There are a couple obvious reasons we can’t. Obvious in hindsight.
The number one reason is that when we predict the movement of stars or moons, we don’t change their movements. But when we predict people—and we actually funnel them and channel them into different slots depending on what their score was—or we give them different Facebook feeds depending on what we think they’re going to like, we’re not just predicting what they’re going to like, we’re actually influencing what they’re going to like. So we’re engendering feedback loops. That’s one thing.
The other thing is that we have this belief—which is just wrong—that data itself is inherently objective. That it is somehow created in an objective manner. And in the cases of predictive policing, or recidivism risk algorithms, when the data itself is so completely biased, every single problem of that system follows from the data bias.
We could talk endlessly about what it is we’re doing when we give someone a high risk of recidivism and then send them to prison longer based partly on where they were born rather than what they’ve actually done. But at the end of the day, what we’re talking about is biased data. And it’s biased again because of systemic biases, systemic racism, et cetera. This idea that we’re just going by the numbers, that we’re just following the data, and that the data never lies—that is just wrong.
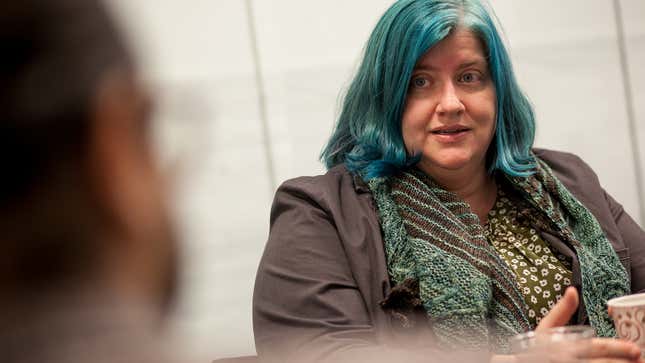
QZ: Let’s look at the case of a hiring manager trying to find an employee. Your book talks about how many such decisions are now being made by algorithms. Should we be comparing the algorithmic decision to some kind of ideal that we’ve thought through ethically and philosophically, or should we be comparing it to what was done previously based on human intuition and interviews?
O’Neil: It’s absolutely a great point that many of these algorithms, although problematic, might actually be improvements from the past. Something like the recidivism risk algorithm—that’s been introduced because judges are nefariously and famously racist. There’s so much evidence that they’re racist. My problem right now is twofold. Number one, these algorithms are also racist. Number two, as far as I know, we haven’t actually measured whether it’s better or worse when you use these scores. And there’s an actual avoidance of answering this question.
So while you’re right that the things that these algorithmic systems are replacing were not perfect, we actually don’t know if it’s getting better or worse. At the very least, let’s see. Let’s test that. Because if we had evidence that judges were racist, we can look for evidence that these new systems are better.
But the other thing I want to say is, the promise of big data, which is not being realized right now, is that we could actually make things better. Plain old better. And we could sit down and have philosophical disagreements and arguments and then sort out some kind of compromise that would involve ideas and theories, and we could implement those using algorithms. And we could have scoring systems that we agree are fair and better than the average stupid human version. But we’re never going to get there if we pretend that what have right now is already perfect.
QZ: One of the things that I’m really freaked out about from reading this book is this idea of algorithms codifying the past. Another point of this question of “is the algorithm better than humans or not” is, well at least in the past, we just knew that society could somehow free itself of racist individuals at some point.
O’Neil: Yeah, they would die out.
QZ: Right. But now we have this model that is thought to be “real” and “objective.”
O’Neil: It’s thought to be a solution to racism, but in fact it’s codifying racism.
QZ: So can you talk about how this process of codifying the past happens?
O’Neil: Yeah. The thought experiment I like to give sometimes is, look at Roger Ailes, if your readers are uncomfortable with the idea of him being guilty as sin of making it impossible for women to succeed as Fox News Anchors. Or just make up any company that has a problem whereby a certain population—say women—are systematically denied success. Not because they’re not good at what they do, but because it’s just a crazy place. And then this happens for 20 years. Now there’s a scandal, Roger Ailes is kicked out, and Fox News vows to do better.
So they introduce a machine-learning algorithm that’s going to replace their hitherto scandalously terrible hiring algorithm. That sounds like good news for people who haven’t read my book, because they’re like, “Oh, machine learning, that’s going to make it fair.” The problem is, if you think about what that means, you’re going to have 20 years of data on people applying to Fox News, and ask, “Ok, five years after that did they get promoted? Did they stay for five years?”
As a data scientist, that’s the kind of thing I’d do. Define success for an applicant as staying there for five years and getting promoted twice. Then I would train my model to look for people that look like these people who succeeded in the past. That’s how machine learning works. And then I would apply that to the current pool of applicants, and I would see that I was systemically removing women. Because women in the past were not allowed to succeed. That for me is a crystalline example of how machine learning codifies the past. As long as you’re training it on historical data that is problematic.
QZ: Right, and we don’t have any other kind of data to train it on.
O’Neil: We don’t. We really don’t.
QZ: Do you think we give humans enough credit for being able to make good decisions?
O’Neil: We as a society or as data scientists?
QZ: I think I’m looking at the societal level. Personally I think, if we have systems that we know are unfair or unequal, and this is known by humans in society, it’s better to just have humans make decisions with the knowledge of this unfairness Versus trying to create some abstract model that ends up codifying these biases forever.
O’Neil: I really think it depends. So, I actually think that one of the things that’s great about humans is that if you had a bunch of human hiring managers, they would have different biases. And one of the problems that I talk about in the book is that, with the algorithm, the same mistake is propagated throughout the system.
Let me give you an example where I really do feel like we are trusting the machines too much. This is when it’s not systematic at all. I feel like, Nate Silver fucked up with Donald Trump for a really important reason, which is that Trump was successful in a way that Nate Silver didn’t recognize. Even when he was polling well, Nate Silver dismissed him, because he said, I’ve never seen this kind of success.
For that same reason—I hope you can jump with me over here—the machine learning algorithm is never going to figure out who’s the next great painter. The algorithm might very well be able to tell you whether a certain new painting or painter is going to be relatively successful, because it looks a lot like successful paintings. But it will never be able to say, this is new, and it’s awesome. And that is fundamentally the job of a human being, to say, this is new, and it’s a big deal.
So that’s an argument from my book, which is that algorithms will never improve us as a society. Because the best they will do is help us create something that we’re comfortable with and that we think of as fair. But as we evolve, they will never keep up with us. We will have to explicitly evolve the computers. We’ll have to train the algorithms. There will always be a lag between even the best, most fair and most principled algorithm and the humans that are leading the way. We are leading the way.
QZ: What can we do to make sure that we’re using math, machine learning, and data science to create the society that we want versus baking in the one that we have right now, or potentially making it worse?
O’Neil: There’s a lot we can do in the direction of making sure the algorithms we’re using are forces for good rather than forces for evil. We haven’t even begun to consider this. We certainly haven’t required algorithms to undergo these “safety checks,” as I call them.
We’re at the stage where we were probably 100 years ago or more, when car companies put out cars that like, had wheels falling off. And we’re just like, “Oh! Ok, you died.” We need to define safety standards, and check on them as we do with things like cars. It’s not a perfect analogy because the truth is, at least everyone notices when you die in a car. The neighbors notice. Whereas with algorithms it’s not as apparent that people have suffered. So maybe a better analogy would be like, we used to let people pollute rivers. But now we have this idea that this is toxic, and you need to keep track of it.
But we’re starting to pay more attention to it. We have no definitions of safety standards, we have not instituted them, and we need to start doing that. The good news is, we can totally do that. We can totally do that. We need to build tools to do that.