Some of Google’s top AI researchers are trying to predict your medical outcome as soon as you’re admitted to the hospital.
A new research paper, published Jan. 24 with 34 co-authors and not peer-reviewed, claims better accuracy than existing software at predicting outcomes like whether a patient will die in the hospital, be discharged and readmitted, and their final diagnosis. To conduct the study, Google obtained de-identified data of 216,221 adults, with more than 46 billion data points between them. The data span 11 combined years at two hospitals, University of California San Francisco Medical Center (from 2012-2016) and University of Chicago Medicine (2009-2016).
While the results have not been independently validated, Google claims vast improvements over traditional models used today for predicting medical outcomes. Its biggest claim is the ability to predict patient deaths 24-48 hours before current methods, which could allow time for doctors to administer life-saving procedures.
The biggest challenge for AI researchers looking to train their algorithms on electronic health records, the source of the data, is the vast, disparate, and poorly-labelled pieces of data contained in a patient’s file, the researchers write. In addition to data points from tests, written notes have traditionally been difficult for automated systems to comprehend; each doctor and nurse writes differently and can take different styles of notes.
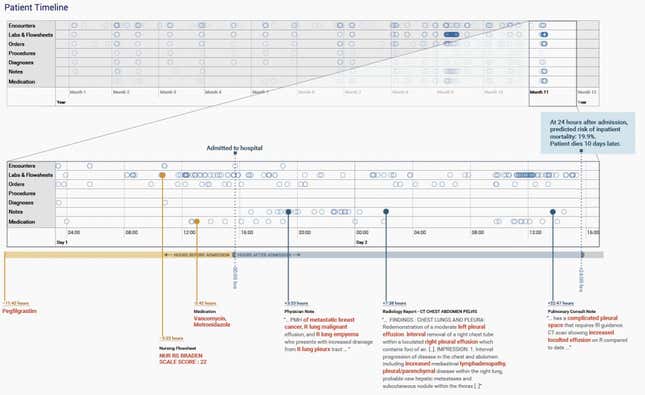
To compensate for this, the Google approach relies on three complex deep neural networks that learn from all the data and work out which bits are most impactful to final outcomes. After analyzing thousands of patients, the system identified which words and events associated closest with outcomes, and learned to pay less attention to what it determined to be extraneous data. Typically, AI scientists have to carefully tinker with how their system interprets the data after it’s built, like which number of layers are needed to make the decision most accurately. In the research paper, the authors write that this was done automatically by a previous Google project called Vizier.
Beyond the paper’s results, the research represents a considerable investment in applying AI to health, outside of Alphabet’s established companies like Verily, Calico, and DeepMind. Google heavy-hitters like Quoc Le, credited with creating recurrent neural networks used for predictions based on time, and Jeff Dean, a legend at the company for his work on Google’s server infrastructure, are both on the paper, as well as Greg Corrado, a director at the company involved in high-profile projects like translation and its Smart Reply feature.
The specialized technology also could threaten work from companies like IBM, which has pitched itself as an innovator in medical AI, but received backlash for making big promises with little tangible results.
Google did not immediately respond to Quartz’ questions.