Why bad data make bad movies
This article is based on a chapter from An Economist Walks into a Brothel, a new book from Quartz economics reporter Allison Schrager.
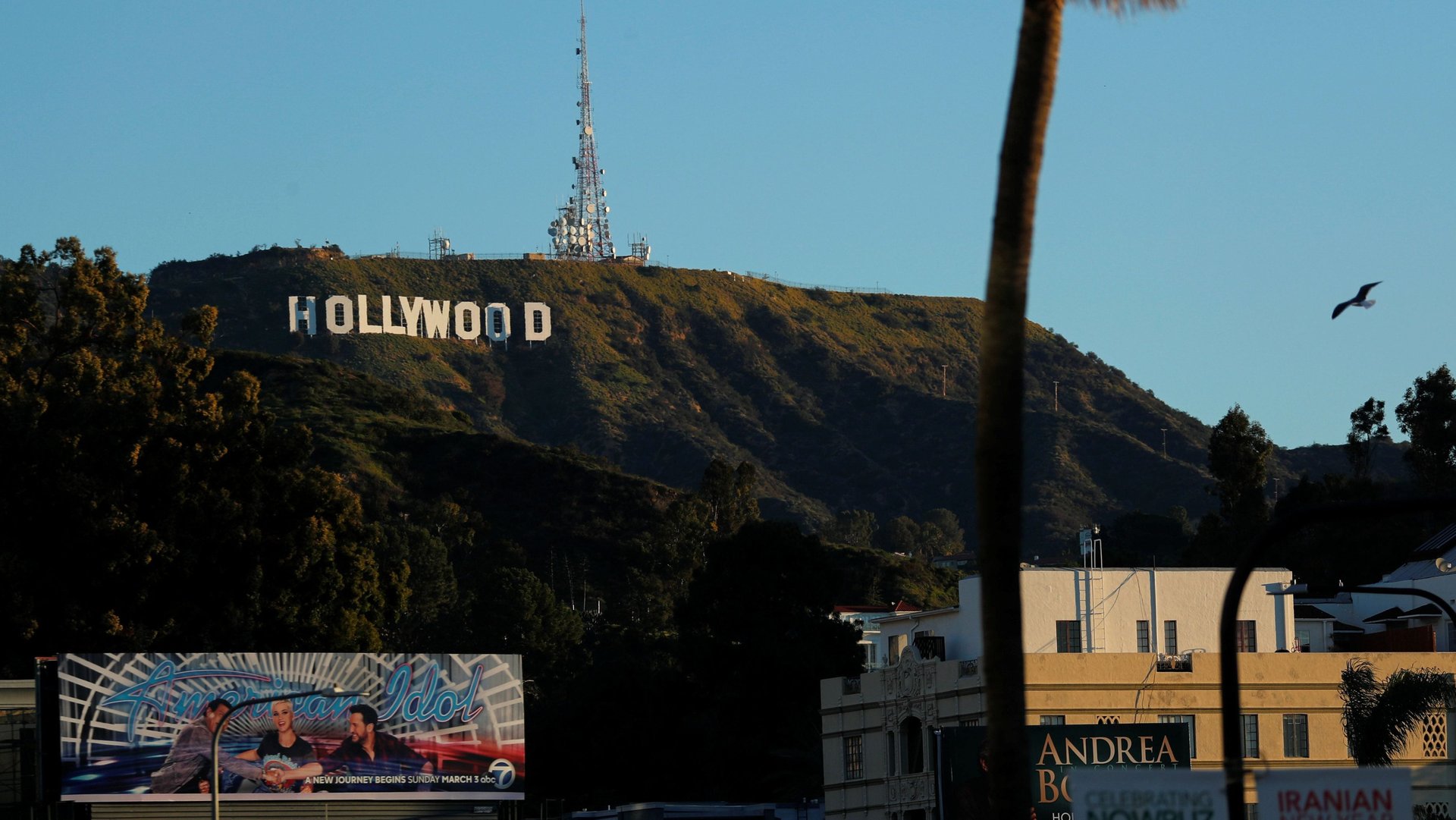
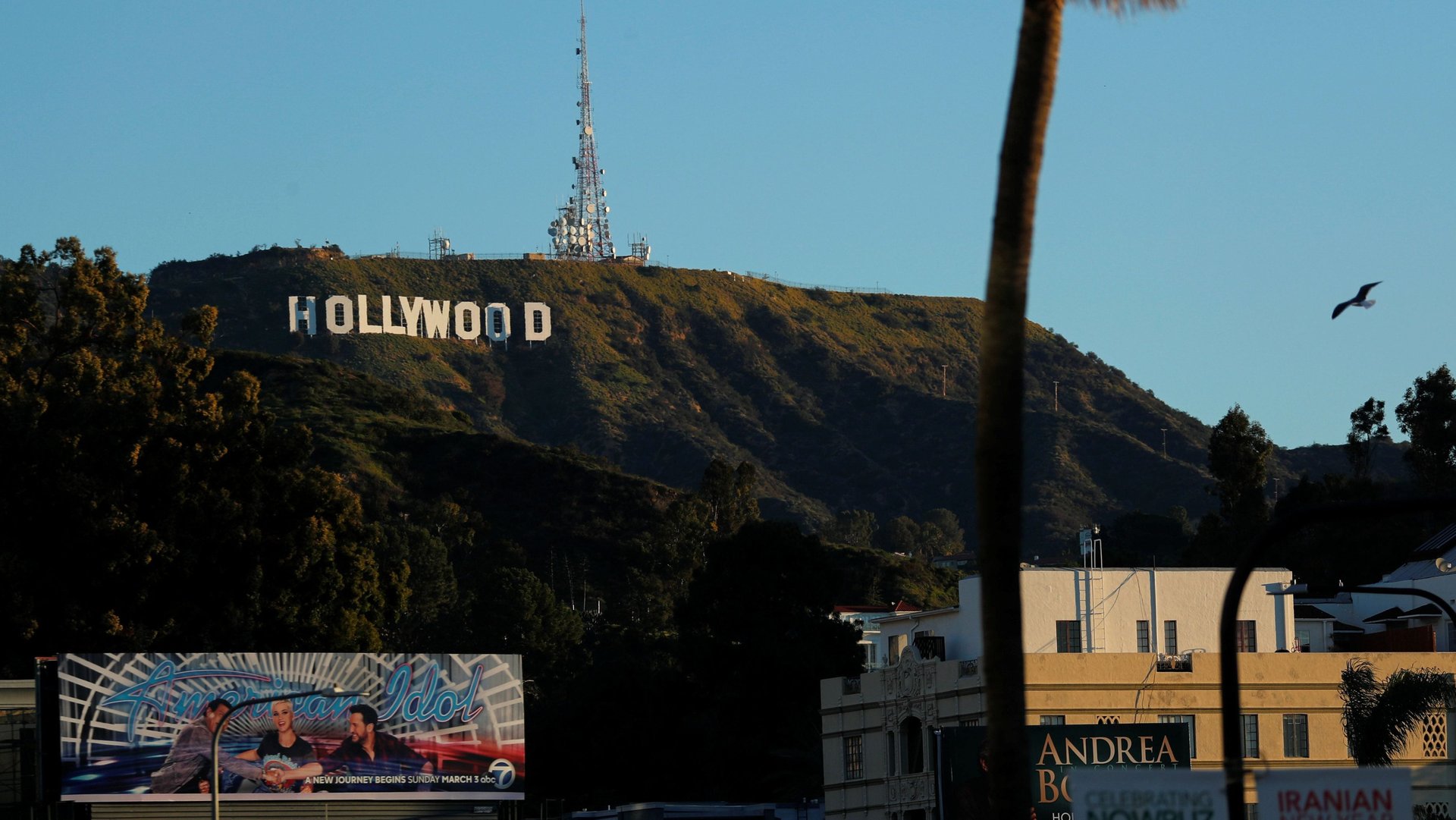
This article is based on a chapter from An Economist Walks into a Brothel, a new book from Quartz economics reporter Allison Schrager.
Hollywood is often called the land of broken dreams. Every day young, talented people come to Hollywood hoping to make it big, only to leave with bitterness and regret. Hollywood could also be called the land of broken risk models. Investors, including banks, hedge funds, and insurance companies, have a long history of coming to Hollywood thinking they can tame the market with science and data. Those dreams also can end in tears—and lawsuits.
A recent casualty is Ryan Kavanaugh, a Los Angeles native who charmed Hollywood with talk of his Monte Carlo simulation—an economic forecasting technique—that lived in an elaborate Excel spreadsheet and promised to make the unpredictable predictable. He claimed his model could forecast which movies would do well and which ones would bomb. It was a seductive pitch.
People in the movie business explain that it is impossible to forecast what will be a blockbuster or a flop. Each film is like a small business with hundreds of moving parts, all of which impact the quality of the film. Because you can’t predict the vagaries of audience taste, the only way to manage risk is to make lots of movies. Most won’t make money, but a few will hit it big and pay for the others. This is a risky way to run a business, and it also explains why there are so many bad movies, with terrible, derivative plots, that fail at the box office. Every year brings both a notorious clunker that cost hundreds of millions to make and an independent drama, with a great script, that cost only $10 million and earned $300 million.
If someone could come up with a way to scientifically pick winners, then a well-functioning moviemaking market would be ripe for the taking. Enter Kavanaugh.
He cofounded Relativity Media in 2004 before he turned 30. Armed with a team of number crunchers, he marketed himself as a math whiz in jeans who could provide the predictability Hollywood craved. His timing couldn’t have been better because movie studios needed a new source of financing in the mid 2000s. For years they had depended on a German tax shelter to attract investors and off-load the considerable financial risk involved with making movies, even if they weren’t filmed in Germany. But Angela Merkel’s coalition government nixed the shelter after she took office in 2005.
Losing the German tax shelter left studios unsure how to get financing. In the meantime, hedge funds were looking to invest in riskier assets with the potential for high returns. It was a perfect match. Kavanaugh jumped at the opportunity, especially since hedge funds, with their roots in finance, have to quantify any risk they take.
He selected certain movie characteristics (such as actor, director, genre, budget, release date, and rating) and estimated which ones would become a winner in the future by analyzing data for the same inputs from previous films. The model produced a range of potential profits based on how these characteristics had performed in the past.
Kavanaugh claimed his model could produce a range of reliable potential earning scenarios for any given film. If more than 70% of those earning scenarios were associated with enough profit, Kavanaugh advised investors to invest in the film as part of a slate of movies he handpicked. Studios were so enthusiastic about the potential for financing that they shared full data on their profits with Kavanaugh, which he called the “Holy Grail” of Hollywood.
Hedge funds and banks plowed hundreds of millions of dollars into the movies Kavanaugh picked. In 2005 and 2006, he financed 36 movies, including The Social Network and Atonement, with Universal and Sony and made money for his investors. Hedge fund investors earned a $150 million profit on one of his early slates, a return of between 13 percent and 18 percent. Kavanaugh was paid millions of dollars per movie and got a producer credit despite having no role in production.
But then Kavanaugh got greedy. Elliott Management, a $21 billion hedge fund, paid $67 million for 49.5 percent of Relativity in 2008. This gave Kavanaugh access to the money he needed to start investing in movies himself. His spending got out of control: he brought exotic animals into the office; and he started to work out of a lavishly decorated airport hangar. Worse for investors, his magic model stopped working, and he selected bombs like The Warrior’s Way, which cost $42 million to make and brought in $5.7 million in the US, and Machine Gun Preacher, with domestic earnings of only $539,000. After Elliott Management pulled out in 2010, Kavanaugh managed to find more financial backers, but continued to struggle as his spending accelerated and he picked more duds. Relativity was bankrupt by 2016.
Once again, Hollywood broke a risk model.
Risk measurement
The future is always uncertain, and we can’t know which movie will be a blockbuster and which will flop. We don’t even know exactly how long it will take to drive to the airport. All we can do is take a guess, usually based on data about what happened in the past. It could be the last 10 trips to the airport, or in Kavanaugh’s case, past movie successes and failures. Data is the best we’ve got, but it’s a terrible way to predict the future.
It is easier to make decisions when can make an accurate risk measurement, or decent prediction of all the things that might happen in the future. If you know from previous trips it takes between 35 and 40 minutes to get to the airport, predicting an accurate travel time is pretty simple. Not so if it could take between 15 minutes and 3 hours. Whether a risk is easy to measure or not comes down to the quality of the data, and whether you can find patterns in it that tell you something.
Movie data is especially hard to work with because the market keeps changing. Fifteen years ago, DVD sales were a solid source of revenue and now that market has all but disappeared. A bad ranking from Rotten Tomatoes can undermine even the best marketing strategy, a factor that didn’t exist a decade ago. In recent years, comic-book franchises have come to dominate the market, in part because Chinese movie goers have became a bigger source of revenue. Movie insiders I spoke to expect this trend to play out eventually, too. Anyone with longevity in the business has seen many fads come and go.
The other challenge is the nature of the data. Risk measurement requires data that can tell you how likely some events are to happen. For example, if past data tells us it will probably take between 20 and 40 minutes to get the airport most of the time, you can safely ignore the possibility of a three-hour trip. Movie data doesn’t offer such reliable estimates. Most movies lose money at the box office and among those that make money, success can range from barely breaking even to a blockbuster. Everything in the profit range is equally likely. It is what financial modelers call a skewed distribution, and it’s what makes risk measurement, in movies or in other domains like investing in start-ups, so hard.
This is why models like Kavanaugh’s are doomed to fail and why Hollywood tends to double down on predictable cinematic trends and has historically ignored the tastes of potentially lucrative audiences like women and minorities. Trends offer a momentary bit of certainty, while betting on an unproven audience feels like long shot. Odds are a Marvel movie will make money, for now.
The promise of big data
Bad data has a long history in Hollywood. Kavanaugh was not the first to try to measure the unmeasurable and he won’t be the last. But with big data—data built of many observations— the next generation may be more successful. Netflix can give you a recommendation based on the likelihood that someone with your demographic profile finished watching that movie. Instead of making risky predictions based on a rough estimate from your past—and limited—moviegoing experience, you can make decisions based on the experiences of millions of other movie goers.
Big data may not only help you make better viewing choices, it can also transform the movie business. More data means more accuracy. Machine learning can also help spot patterns so it will be possible to know which data is useful and what’s out of date because the technology can identify structural breaks when data is no longer useful. Amazon and Netflix have data on precisely who watches what and whether they finish watching the movie, a useful proxy for whether they liked it. Currently, almost half of a movie’s budget is spent on marketing because films are advertised to everyone, with the vague hope the advertising will appeal to someone. Now that studios have more data on viewing patterns, they can better tailor their marketing strategy and know which movies will appeal to their intended audience. This means studios could spend less on marketing freeing them to take more risks elsewhere.
Better data might mean fewer fads, better movies, and more predictability for the business and viewers.