Customers like to shop. They do not, however, like to wait.
This unsurprising discovery, Amazon vice president Dilip Kumar said, is at the heart of Amazon Go’s in-person retail stores, in which customers simply select their goods of choice, place them in their bag, and go. No lines, no check-outs, no problems. (There are currently 12 stores across the US.)
But such a “magical” shopping experience doesn’t come easy. Speaking at Amazon’s inaugural public Re:MARS conference on robotics, machine learning, automation, and space today, Kumar laid out the three problems Amazon Go engineers faced in producing staggeringly twiddly technology to solve these comparably complex problems. (The sensor- and AI-powered technology is similar to what makes self-driving cars work: computer vision, merging data from multiple sensors, and deep learning.)
The first problem, Kumar said, was using computers and sensors to figure out which Amazon Go customer account to charge items to. From there, he said, “in order for us to generate accurate receipts, we needed to keep track of the interactions that you have with the products and associate those interactions to the nearest customer account.” This might sound easy enough, but it was made harder by the random movements of customers (as well as any stragglers who might be along for the ride), which the computer tracked as they moved about the store.
The second problem arose in working out what, precisely, these shoppers were actually taking from the shelves, then charging them appropriately. To begin with, products that look very similar—different jars of peanut butter, for instance—tend to be placed side by side on the shelves. Soft packaged goods may warp when they’re picked up; shiny packaging reflects sunlight, obscuring the information on the label. Then, of course, there are the issues introduced in the normal course of shopping—a splayed hand hiding the label, say, or a fast-moving grab introducing motion blur to the footage.
While some of these problems might have been avoided by asking customers to hold the package up to the camera, this seemed contrary to the spirit of Amazon Go stores, Kumar said, in which customers should shop as naturally as possible.
To solve these two issues, machine learning algorithms had to be trained with huge volumes of data—including errors. Without a bank of information to turn to, the company instead generated fake errors—footage of fake, animated people picking products within the store; networking blips; camera outages—to teach the algorithms to understand how to understand what was going on.
Eventually, Kumar said, the machines were able to deduce who was entering, who was leaving (and with whom), and all the other kinds of “retail scaffolding”—what products, how much they cost, and so on and so forth.
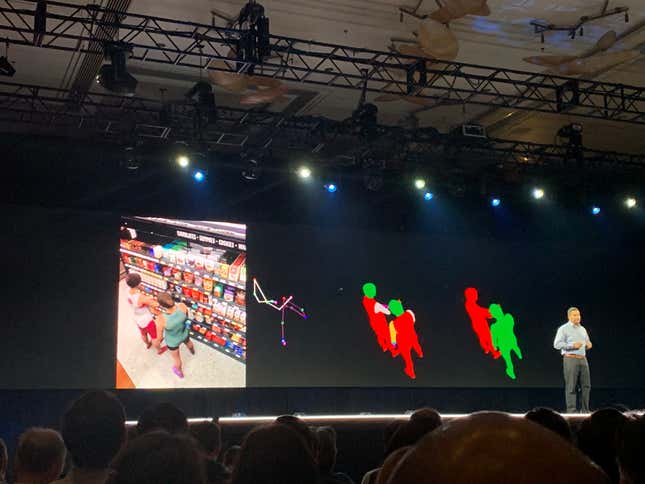
But while these two problems were hard enough on their own, there was a third that was still more difficult, Kumar said: how to take “this entire complexity” and make the tech recede into the background, so that customers could simply shop and go.
A big ask—but worth the effort, Kumar said. “Amazon Go is a very practical example of AI. You can use the power of computer vision to build a stellar customer experience that your customers love.”
Assuming they have an Amazon Go account and an active credit card, that is.