The smart guesswork that applies even in Nate Silver’s world
At 12:13 a.m. on Nov. 7, Nate Silver tweeted: “This is probably a good time to link to my book: http://tinyurl.com/andexhw.” Sent about an hour after the networks had called the election for President Barack Obama, the tweet had by the time of this writing been favorited over 2,500 times and retweeted nearly 7,000 times. The FiveThirtyEight blogger at the New York Times had silenced his critics with a dead-on forecast of the presidential election, correctly predicting which candidate each of the 50 states would vote for—surpassing even the 49-out-of-50 performance that made his name in the 2008 election. He was right about his sense of book promotion as well—The Signal and the Noise vaulted to No. 2 on the Amazon rankings, where it has sat since. Even Amy Chua’s 2011 blockbuster Battle Hymn of the Tiger Mother reached only No. 4 on Amazon at its height.
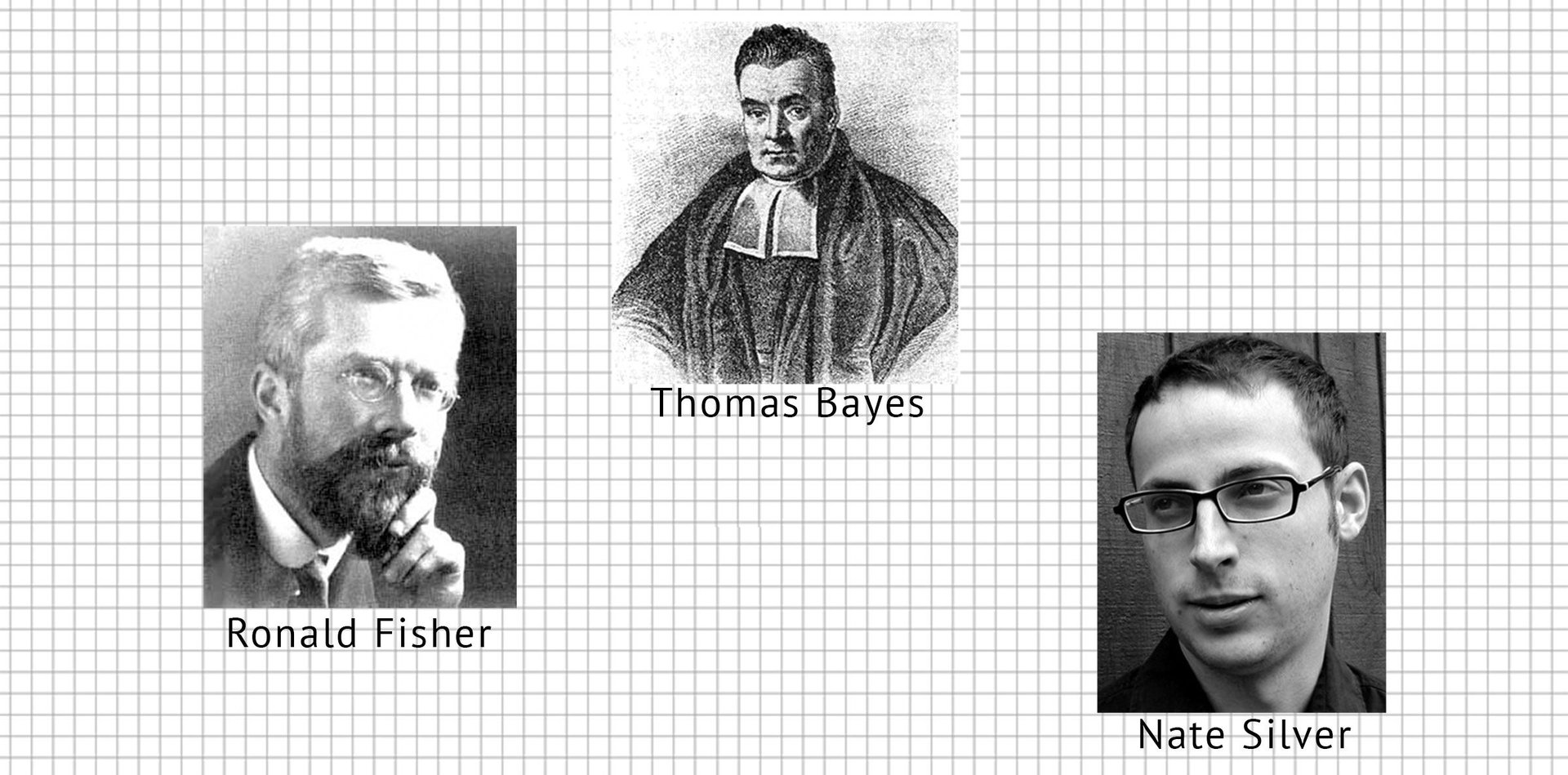
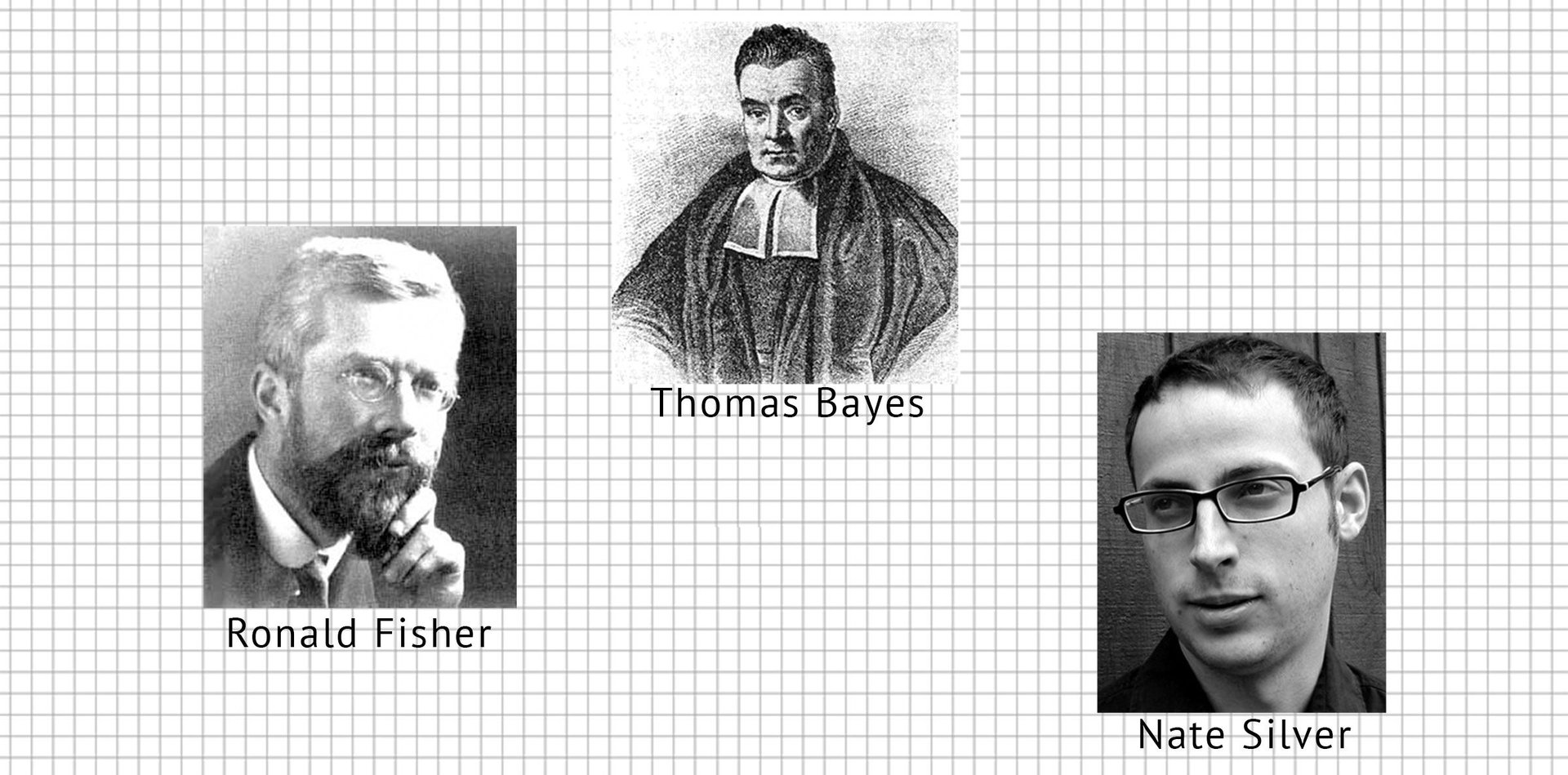
At 12:13 a.m. on Nov. 7, Nate Silver tweeted: “This is probably a good time to link to my book: http://tinyurl.com/andexhw.” Sent about an hour after the networks had called the election for President Barack Obama, the tweet had by the time of this writing been favorited over 2,500 times and retweeted nearly 7,000 times. The FiveThirtyEight blogger at the New York Times had silenced his critics with a dead-on forecast of the presidential election, correctly predicting which candidate each of the 50 states would vote for—surpassing even the 49-out-of-50 performance that made his name in the 2008 election. He was right about his sense of book promotion as well—The Signal and the Noise vaulted to No. 2 on the Amazon rankings, where it has sat since. Even Amy Chua’s 2011 blockbuster Battle Hymn of the Tiger Mother reached only No. 4 on Amazon at its height.
The 34-year-old Silver is America’s newest literary star, rising in a way that seems strange in a country that, when it does idolize the brainy, usually goes for a tech titan, a mouthy political pundit or, in Chua’s case, someone resolving one of Americans’ deepest insecurities: how to make their kids succeed. The occasional economist or diplomat may attain some level of fame, but usually only after they are already validated by the Nobel Prize Committee. In Silver’s case, we have a mere self-styled baseball-statistics-fanatic cum politics-maniac, a geek absent any discernible attitude apart from a faithfulness to data, as we found when we spoke with him before his September book launch.
But the flood of admiration has missed an essential point, which is that Silver is not only a politics geek. If you happen to be generally tired of vapid, dumbed-down treatments not just of politics but of finance, poker, the weather, climate change, not to mention sports of many types, Silver’s book applies his methodology to those subjects, too.
As the title of his book suggests, Silver’s thesis is that, to evaluate a situation with many moving parts, you need to pick out the signal—the crucial piece or pattern of data—from unessential, distracting and misleading noise surrounding it. If you do it right, you can glean a reasonably sound probability of a given outcome. But Silver also cautions that in some fields, such as economics and national security, the noise is so thick that forecasts are not very reliable.
The problem in the world of prediction, and the reason so many rivals get their forecasts wrong, posits Silver, is that the very core of their statistical analysis is flawed. They are using a method called “frequentism,” which judges probability purely by the frequency of events in a statistical sample. It was developed by, among other thinkers, a fellow named Ronald Fisher, whom Silver spends some pages undermining. Where Silver departs from Fisher and the other frequentists is that they ignore the context surrounding their statistical sample. What happened before the event they are investigating statistically, for example? What is going on around it?
Silver prefers the methods of a Fisher predecessor named Thomas Bayes. Bayes advocated the use of data collection, too, but demanded the introduction of context in its evaluation. (This comic at xkcd.com is worth looking at.)
Applying Bayes, Silver likes a gambler’s approach. A strict frequentist might play poker by calculating the likelihood that his opponents’ cards are weaker or stronger than the ones he holds, based on the cards that a standard deck contains; or by analyzing thousands of past poker games and calculating how often a certain sequence of bids has led to a victory for one player or the other. But a true poker player (one of Silver’s past professions) includes whatever contextual information he can add. How aggressively or passively have his opponents played in the past given their cards? Are they younger or older? Given how much money they have won or lost, how much more or less likely are they to bluff?
This is not the picture that most of us have after reading Silver’s FiveThirtyEight columns and marveling over his election forecasts (On Nov. 10, he weighed in on which polls fared best in the election). Much of the world seems to think that he is pure grey matter, and not swayed by mere judgment of what is probably correct, or too absurd even to consider.
But Silver’s point is that if having a lot of data were sufficient, there would be fewer mysteries in the world, given the escalation of pure information in our midst. The problem in making reliable predictions is a failure to apply cool judgment to our data. He considers it no coincidence, for example, that political partisanship in America has increased with the rise of the internet; people now have more information, but are using it to reinforce preconceived beliefs, instead of to choose between competing viewpoints. Of the failure to predict the 9/11 attacks, he notes, “The problem was not want of information. As had been the case in the Pearl Harbor attacks six decades earlier, all the signals were there. But we had not put them together. Lacking a proper theory for how terrorists might behave, we were blind to the data.”
To predict an election, therefore, it’s not enough to look at polls and average them out. You need to have a common-sense theory about how other things might alter the accuracy of those polls, and then test that theory using the methods of statistics. For instance, how does the unemployment rate affect polls? What about GDP growth? Was polling done solely to land lines, or to cell phones as well (these days, many people do not have home phones, so pollsters who rely solely on robo calls to land lines may produce inaccurate projections)?
Silver’s record comes in identifying such outside factors to throw in the stew with the nation’s polls, and determining how much weight to assign to each. The purist Fisher would have ignored the indirect data, and stayed with the poll results. Perhaps he still would have produced the correct election forecast, but Silver suggests he would have had a better chance doing so under Bayesian methods.
Fisher, says Silver, could not reliably distinguish between signal and noise.