You can measure illiteracy around the world just by looking at mobile phone data
Illiteracy takes a massive toll on peoples’ lives, as well as $1.2 trillion out of the global economy each year. People unable to read are shut out of jobs, services, and wider social networks, trapping them in poverty. As the developing world moves online, this disadvantage will only grow steeper.
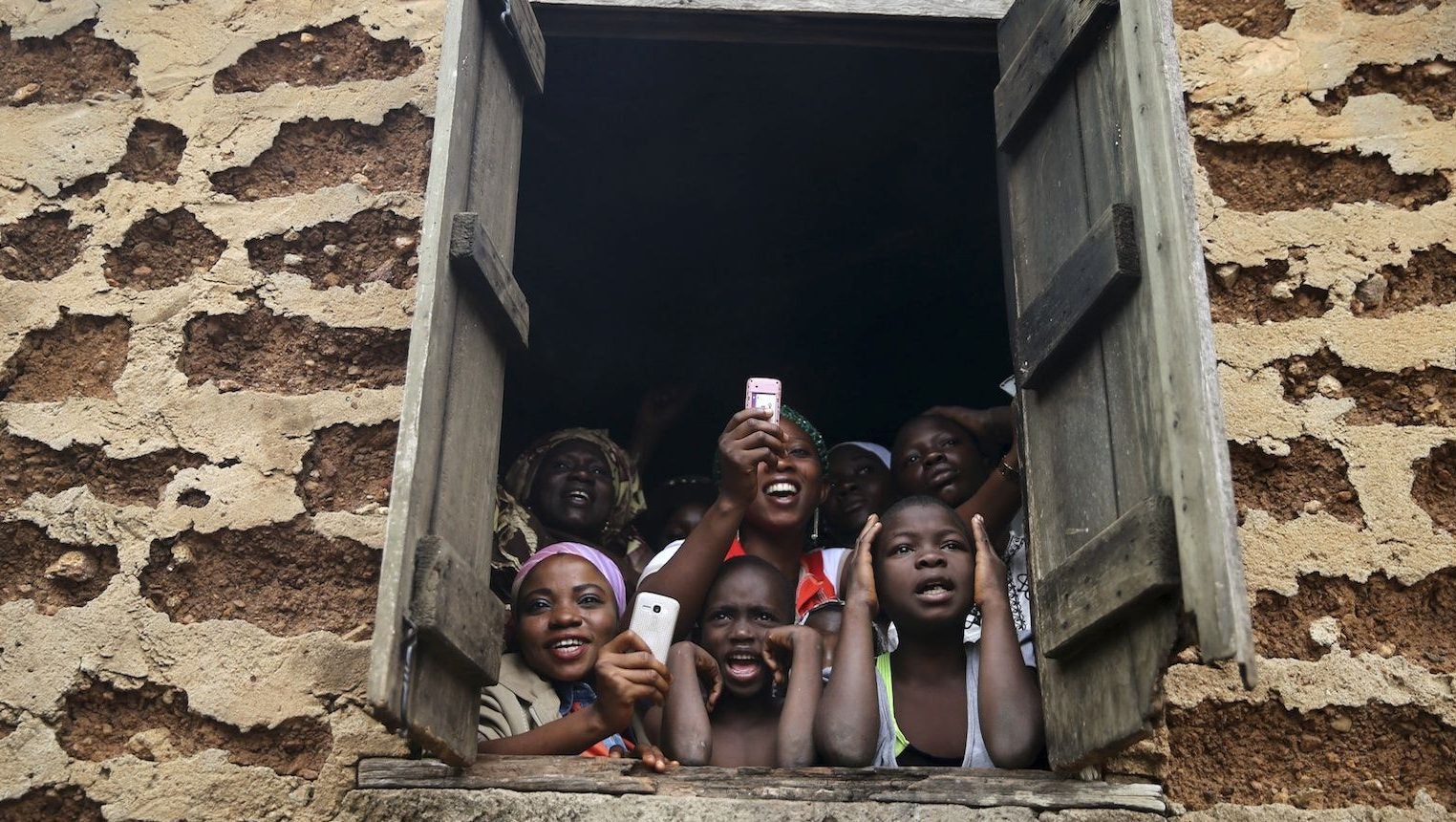
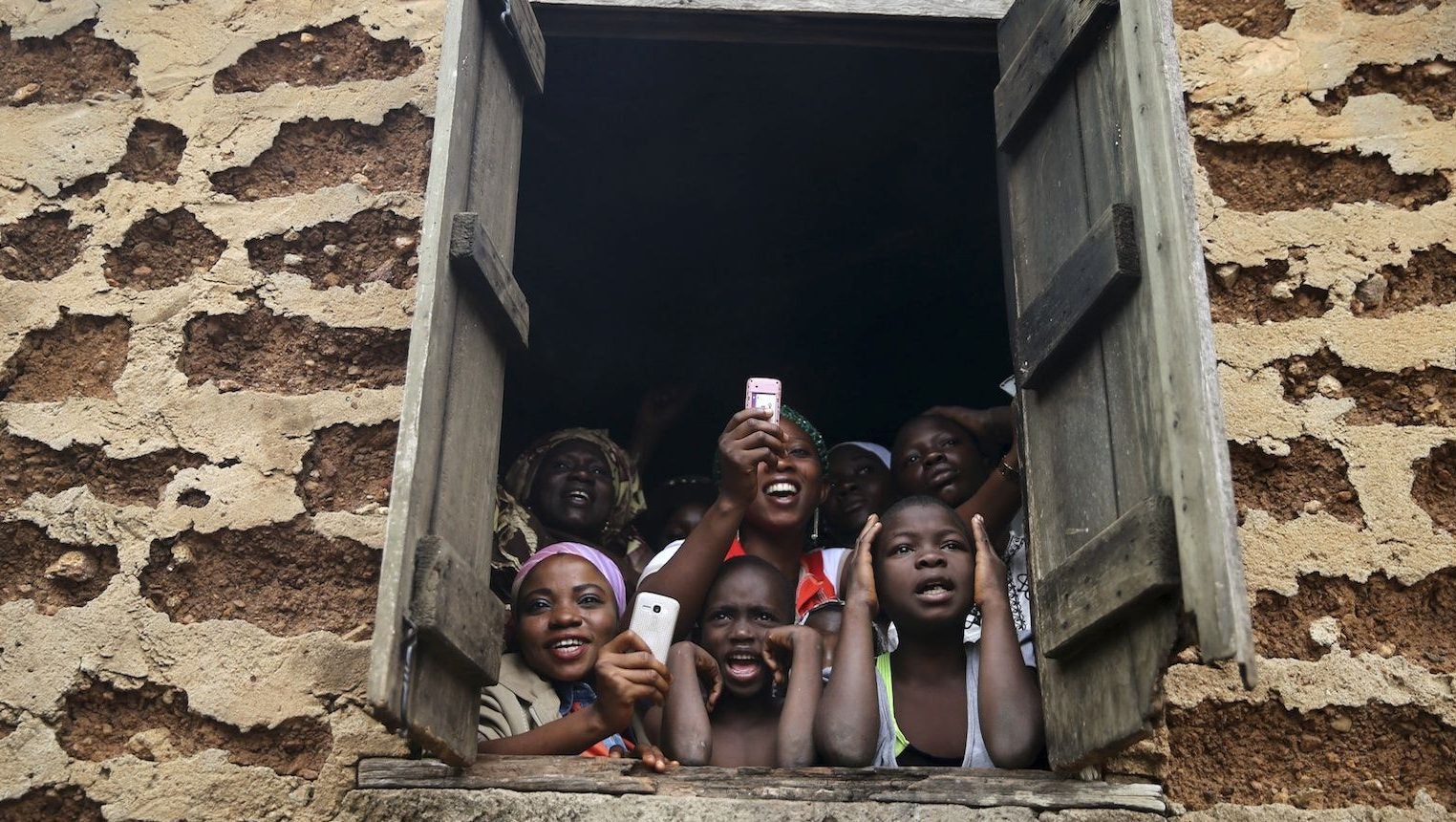
Illiteracy takes a massive toll on peoples’ lives, as well as $1.2 trillion out of the global economy each year. People unable to read are shut out of jobs, services, and wider social networks, trapping them in poverty. As the developing world moves online, this disadvantage will only grow steeper.
Part of the challenge is gathering detailed information about the problem so it can be effectively targeted and progress assessed. This can be dauntingly expensive for a social issue like illiteracy. Morten Jerven, a professor at Canada’s Simon Fraser University, estimates properly monitoring just 18 targets for the United Nations’ Millenium Development Goals will cost $27 billion. In some countries, data on social indicators is hopelessly out of date or isn’t collected at all due to the expense and expertise needed to collect it.
Norwegian data scientist Pål Sundsøy at the Telenor Group Research released a paper on arXiv last week showing how machine learning can turn mobile phone records into a high-resolution map of illiteracy in developing countries. The research predicted illiteracy rates with 70% accuracy from users’ mobile phone data and mapped it.
The approach, once tested at scale, could give governments a much more cost-effective way to track and address illiteracy rates in areas where there is little data. While survey data is scarce and expensive in poor countries, mobile phones are not. Of the 10 countries with the lowest literacy rates according to the UN, all but two have a higher percentage of the population with at least one subscription to a mobile phone service.
Geography (as tracked via individual cell towers) and ratio of incoming versus outgoing SMS messages were found to be the most highly correlated with illiteracy. Illiterate users tended to live and travel in small areas, and exchange less communication with their contacts, particularly over text. Their social networks were also smaller and less active than the rest of the population. “This signal indicates that the model [can] catch regions of low economic development status, e.g. slum areas where illiteracy is high,” writes Sundsøy, who reportedly pinpointed three large pockets of high illiteracy rates in a study city.
The study analyzed the phone records of 76,000 people in a low-income country in Asia. Existing literacy surveys served as a baseline. Algorithms sifted through anonymized data on phone models, travel patterns, social networks, voice and data usage, and credit purchases to pick out the most important factors.