Apple has long been secretive about the research done within its Cupertino, California, labs. It’s easy to understand why. Conjecture can spin out of even the most benign of research papers or submissions to medical journals, especially when they’re tied to the most valuable company on the planet.
But it seems Apple is starting to open up about its work, at least in artificial intelligence. On Dec. 6, at an invitation-only lunch at an industry AI conference, the company’s new head of machine learning, Russ Salakhutdinov, and other Apple employees gave a wide-ranging talk detailing the problems the company is using AI to tackle, according to nine slides from the presentation obtained by Quartz.
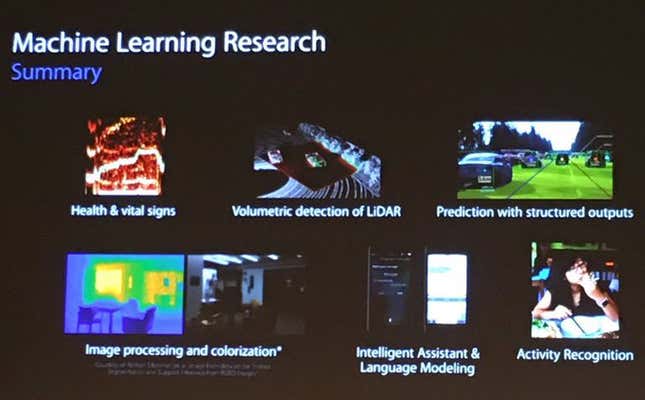
Apple, unsurprisingly, is working on a lot of the same problems as other companies that are exploring machine learning: recognizing and processing images, predicting user behavior and events in the physical world, modeling language for use in personal assistants, and trying to understand how to deal with uncertainty when an algorithm can’t make a high-confidence decision.
One presentation slide that summarized the company’s research featured two pictures of cars, to illustrate “volumetric detection of LiDAR” and “prediction of structured outputs.” Both LiDAR, or Light Detection and Ranging (similar to radar but with lasers), and prediction of physical events are important building blocks of today’s self-driving car technologies. However, two attendees of the presentation, who asked not to be identified due to the sensitive nature of the content, both stressed that the company made no mention of cars or automotive ambitions.
Apple has been long-rumored to be building an autonomous vehicle, even sending a letter to the US National Highway Traffic Safety Administration (NHTSA) saying that companies looking to test autonomous vehicles should be treated the same, whether they are new to the field or more established self-driving car shops like Google and Uber.
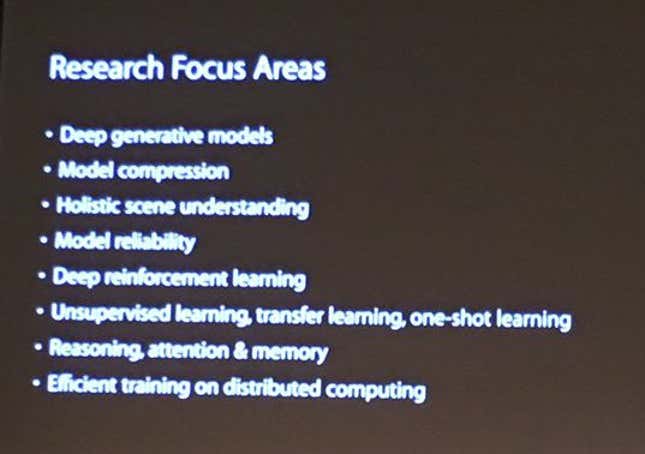
Another slide focused on Apple’s ability to build neural networks that are 4.5 times smaller than the originals with no loss in accuracy, and twice the speed. The technique, not unknown in AI research, uses a larger, more robust neural network to teach another network the decisions it would make in a variety of situations. The “student” network then has a streamlined version of the “teacher” network’s knowledge. In essence, it predicts the larger network’s predictions about a given photo or audio sample.
This kind of work is essential for Apple, as a hardware company that makes mobile devices. By slimming down the neural network, iPhones and iPads can identify faces and locations in photos, or understand changes in a user’s heart rate, without needing to rely on remote servers. Keeping these processes on the phone makes the features available anywhere, and also ensures data doesn’t need to be encrypted and sent over wireless networks.
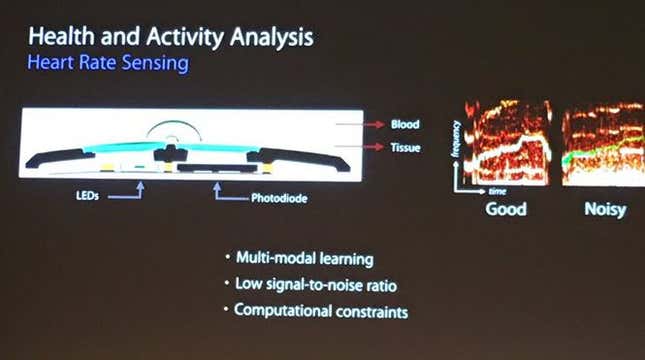
A bragging point for Apple was the efficiency of its algorithms on graphics processing units, or GPUs, the hardware commonly used in servers to speed processing in deep learning. One slide claimed that Apple’s image recognition algorithm could process twice as many photos per second as Google’s (pdf), or 3,000 images per second versus Google’s 1,500 per second, using roughly one third of the GPUs. The comparison was made against algorithms running on Amazon Web Services, a standard in cloud computing.
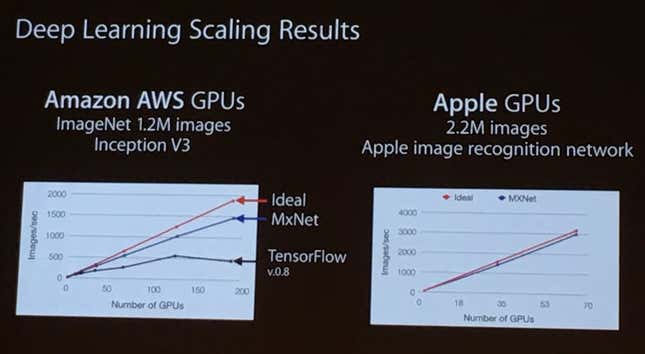
While other companies are beginning to rely on specialty chips to speed their AI efforts, like Google’s Tensor Processing Unit and Microsoft’s FPGAs, it’s interesting to note that Apple is relying on standard GPUs. It’s not known, however, whether the company builds its own, custom GPUs to match its custom consumer hardware, or buys from a larger manufacturer like Nvidia, which sells to so many internet companies it has been described as “selling shovels to the machine learning gold rush.“
The images Apple uses to train its neural network on how to recognize images also seems to be proprietary, and is nearly twice the size of the standard ImageNet database.
Machine-learning scientists have long-criticized Apple for its reluctance to contribute back to the research community. During the presentation, which served to bring a small, select group of researchers up to speed with Apple’s efforts, Salakhutdinov—a prominent AI researcher himself, at Carnegie Mellon University—said that Apple would begin to publish its research and make a greater effort to work with the research community, according to attendees.
It’s unclear whether Apple’s pledge to publish will apply only to machine-learning research, or to other areas of computer-science research, like security.
Apple did not respond to a request for comment.