On the surface, Wilhelmina Ndapewa Onyothi Nekoto and Elfriede Gowases seem like a mismatched pair. Nekoto is a 26-year-old data scientist. Gowases is a retired English teacher in her late 60s. Nekoto, who used to play rugby in Namibia’s national league, stands about a head taller than Gowases, who is short and slight. Like nearly half of Namibians, Nekoto speaks Oshiwambo, while Gowases is one of the country’s roughly 200,000 native speakers of Khoekhoegowab.
But the women grew close over a series of working visits starting last October. At Gowases’s home, they translated sentences from Khoekhoegowab to English. Each sentence pair became another entry in a budding database of translations, which Nekoto hopes will one day power AI tools that can automatically translate between Namibia’s languages, bolstering communication and commerce within the country.
“If we can design applications that are able to translate what we’re saying in real time,” Nekoto says, “then that’s one step closer toward economic [development].” That’s one of the goals of the Masakhane project, which organizes natural language processing researchers like Nekoto to work on low-resource African languages.
Compiling a dataset to train an AI model is often a dry, technical task. But Nekoto’s self-driven project, rooted in hours of close conversation with Gowases, is anything but. Each datapoint contains fragments of cultural knowledge preserved in the stories, songs, and recipes that Gowases has translated. This information is as crucial for the success of a machine translation algorithm as the grammar and syntax embedded in the training data.
Linguists, along with AI researchers concerned with language, describe this as the place effect. A model trained on translations rooted in one cultural context (say, Khoekhoegowab translations of US news archives full of references to American food, customs, and place names) will perform significantly worse when used in a different cultural context (like conducting business between an Oshiwambo speaker and a Khoekhoegowab speaker in Namibia). So Nekoto made sure to collect data that was true to Gowases’s everyday life.
“From day one we said, okay, our target for this week should be at least five songs and two traditional processes, or some poems and a recipe,” Nekoto explained. In December, during Namibia’s wedding season, they translated information about how marriages should be conducted. They talked about funeral rites, fashion from the ’80s, and the country’s history under apartheid.
Normally, they did their work on Gowases’s stoep—the porch in front of her house, shaded by trees bearing mangos, guava, and pomegranates, which is open to visiting friends and relatives. But Nekoto recalls a day when a particularly furious storm rolled in and forced them inside. High winds cut power to the house and sent one of Gowases’s fruit trees crashing through the barrier wall surrounding the property. Nekoto told Gowases she was afraid. Gowases held her hand and began to pray.
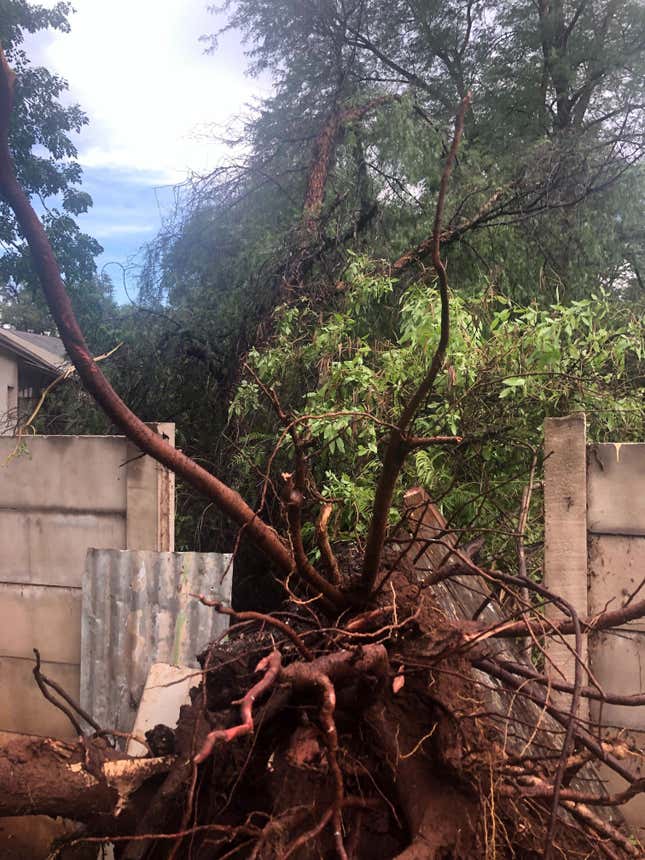
“Ease our fears, for your protection and strength are much greater than our fears,” Nekoto recalls Gowases saying. “Thank you for this rain. May it bring a great harvest that will nourish our souls and keep our reserves full for years to come.”
Before Gowases could finish, Nekoto had her notepad out, and was carefully transcribing every word. “When she saw me writing, she laughed,” Nekoto said. “But then we started coming up with more prayers for various scenarios and wrote those down as well.” As the storm raged on, they worked by flashlight to translate their prayers into English.
This, of course, is a particularly labor-intensive way of building a language dataset. At the end of January, they had translated a few hundred sentences—not enough to train a robust model. Then Nekoto got a long-awaited visa approval to move to Germany and develop an AI system for modeling traffic with HTW Berlin. Separated by thousands of miles and a few technological barriers—Gowases doesn’t use a computer—Nekoto had to come up with a plan to continue their partnership from afar.
Now, Gowases writes out parallel sentences in Khoekhoegowab and English on paper, then hands them off to a computer-savvy collaborator who scans and emails the sheets to Nekoto each week. “Unfortunately, it’s not as easy as other languages,” Nekoto said. “I have to understand that it’s a difficult language. It’s complex, it requires time, and if we had more collaborators we would be able to do more.”
Nekoto does have another collaborator on the data science side—a Masakhane researcher named Musie Meressa, who has helped with a different branch of the project that involves building out a dataset based on a collection of Jehovah’s Witness texts translated into Khoekhoegowab. But so far, Nekoto has done much of the work to collect language data from native speakers by herself.
Still, Nekoto remains optimistic. She says there are more Khoekhoegowab speakers interested now in helping to document their language—especially older retirees who lived through apartheid and want to share their experiences. “We can still collect data from a cultural and historical perspective and continue to build and increase the dataset,” Nekoto said. Little by little, they are growing the collection of digital text available in Khoekhoegowab, and bringing its speakers closer to the AI tools available in the world’s dominant languages.