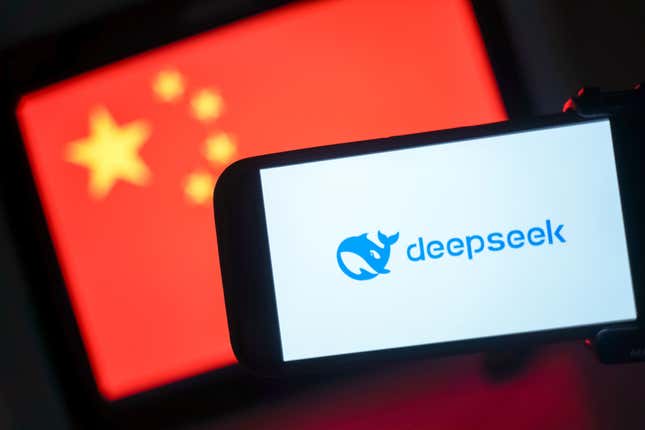
A version of this article originally appeared in Quartz’s members-only Weekend Brief newsletter. Quartz members get access to exclusive newsletters and more. Sign up here.
Not long after ChatGPT came out, a leaked email from Google said what many were thinking but few dared say out loud: “We Have No Moat. And neither does OpenAI.” The May 2023 memo argued that companies would never pay for generative AI when there were open-source options out there – and those models were often better anyway. That same month, halfway across the world, an entrepreneur named Liang Wenfeng quietly founded DeepSeek in China.
A year-and-a-half later, DeepSeek would prove Google prophetic. When DeepSeek revealed its V3 model last December, which the company said it trained for just $5.6 million using inferior chips — less than 6% of GPT-4's training costs — it sent shockwaves through the industry. Then last week, the company unveiled R1, a new reasoning model that can think through complex problems step-by-step, matching the capabilities of OpenAI’s specialized reasoning systems.
These breakthroughs sent American tech stocks into a freefall on Monday and exposed an uncomfortable truth: There might not be any moats in AI at all. The technological barriers that were supposed to protect America’s AI dominance, from advanced chips to massive data centers, are more mirage than fortress. But while these models might spell trouble for companies banking on proprietary advantages or looking for massive funding rounds, DeepSeek could herald a new era of more efficient, accessible AI development.
It wasn’t just companies building generative AI that took a hit. For investors who saw Nvidia as the perfect “picks and shovels” play in an uncertain AI gold rush, DeepSeek’s revelation was also devastating. The company’s stock cratered on Monday, shedding almost $600 billion in the biggest one-day drop in market value in history. It turns out that not only is there no moat for software, as Google warned, but there might not be one for hardware either. That’s jarring for a company whose soaring valuation was built on the idea that AI’s appetite for cutting-edge silicon would only grow.
DeepSeek’s breakthrough came from training its model on about 2,000 of Nvidia’s H800 GPUs — chips that were specifically designed with reduced capabilities to comply with U.S. export controls to China. These are the hobbled cousins of the coveted H100s that American companies use, with deliberately limited chip-to-chip communication speeds that were supposed to make them insufficient for training advanced AI models. Yet DeepSeek managed to create a competitive model despite these constraints.
The advanced chip sanctions put in place by the Biden administration were meant to prevent exactly this scenario. But rather than weakening China’s AI capabilities, the embargo appears to have been the catalyst. DeepSeek was forced to innovate in ways that now challenge Silicon Valley’s fundamental assumptions, even though its founder Wenfeng has acknowledged that the lack of high-end chips remains a bottleneck, according to the Wall Street Journal.
The chip implications go beyond just training costs. When companies find more efficient ways to train AI models, those efficiencies often carry over to how the models run in everyday use — what’s known as inference in the industry. DeepSeek charges $2.19 per million output tokens, compared to $15 for OpenAI’s latest model. That’s not the kind of narrow efficiency gain that can be waved away — it’s a seven-fold difference that threatens to reshape the economics of AI deployment.
Some tech leaders are calling into question whether what DeepSeek did was really possible with its stated budget and chip supply. Meta has reportedly set up “war rooms” to look into these models. Microsoft is probing whether DeepSeek had access to OpenAI tech that could be behind some of its abilities.
If DeepSeek’s claims hold up, it will change the calculus for the frenzied data center build-out across America, including the $500 million Stargate project announced at the White House last week. All these massive facilities felt urgent based on the astronomical costs of training American-made models: OpenAI CEO Sam Altman said GPT-4 cost “more than” $100 million to train, and Anthropic CEO Dario Amodei predicted we could see a $10 billion model this year.
But if they can be trained for a fraction of that cost on less powerful hardware, the rush to build might look more like a costly overreaction. Some, like Meta’s chief AI scientist Yann LeCunn, argue we’ll still need this infrastructure to run AI services at scale. But DeepSeek’s breakthroughs suggest there are still major efficiency gains to be found in both training and deployment, which researchers should be excited about.
It’s a pattern seen again and again.
Just as the cost of computer processing has plummeted since the first mainframes — with smartphones now packing more computing power than the machines that sent astronauts to the moon — there has always been reason to believe AI’s massive energy appetite would come down. The first iterations of any technology are rarely efficient, and the bill for generative AI was always going to come due: Companies need to start making money eventually, and that’s probably impossible at current energy consumption levels.
Or, as Brian Cahn at Sequoia Capital put it, there’s a $600 billion question (which ballooned from his initial $200 billion estimate last summer as AI investments continued to surge while revenue remained elusive) — the gap between what tech companies are spending on AI and what they’re making from it.
DeepSeek’s breakthrough could help close that gap. Since it’s open source, there’s nothing stopping American tech companies from adopting these efficiency techniques themselves. Their own training and inference costs could plummet. And while cheaper AI might seem like bad news for tech giants, Satya Nadella sees it differently. “Jevons paradox strikes again!” Microsoft’s CEO posted on X. “As AI gets more efficient and accessible, we will see its use skyrocket, turning it into a commodity we just can’t get enough of.”
The open-source revelation might mark more than just a technical turning point. The history of AI has shown that the biggest breakthroughs often come from researchers building on each other’s work openly — from the development of neural networks to the transformer architecture that powers today’s AI.
It could reverse what Google researcher François Chollet argued was OpenAI’s biggest impact: setting back artificial general intelligence “5 to 10 years” when it stopped publishing its research, which encouraged less sharing in the field, all in order to protect its advantage.
That moat was never there.
But believing in it may have held back AI more than any technical limitation ever did.