How to tell when you’re being sold pixie dust instead of data science
When I started my data analytics business several years ago, I quickly encountered what I now refer to as the “magic pixie dust” problem.
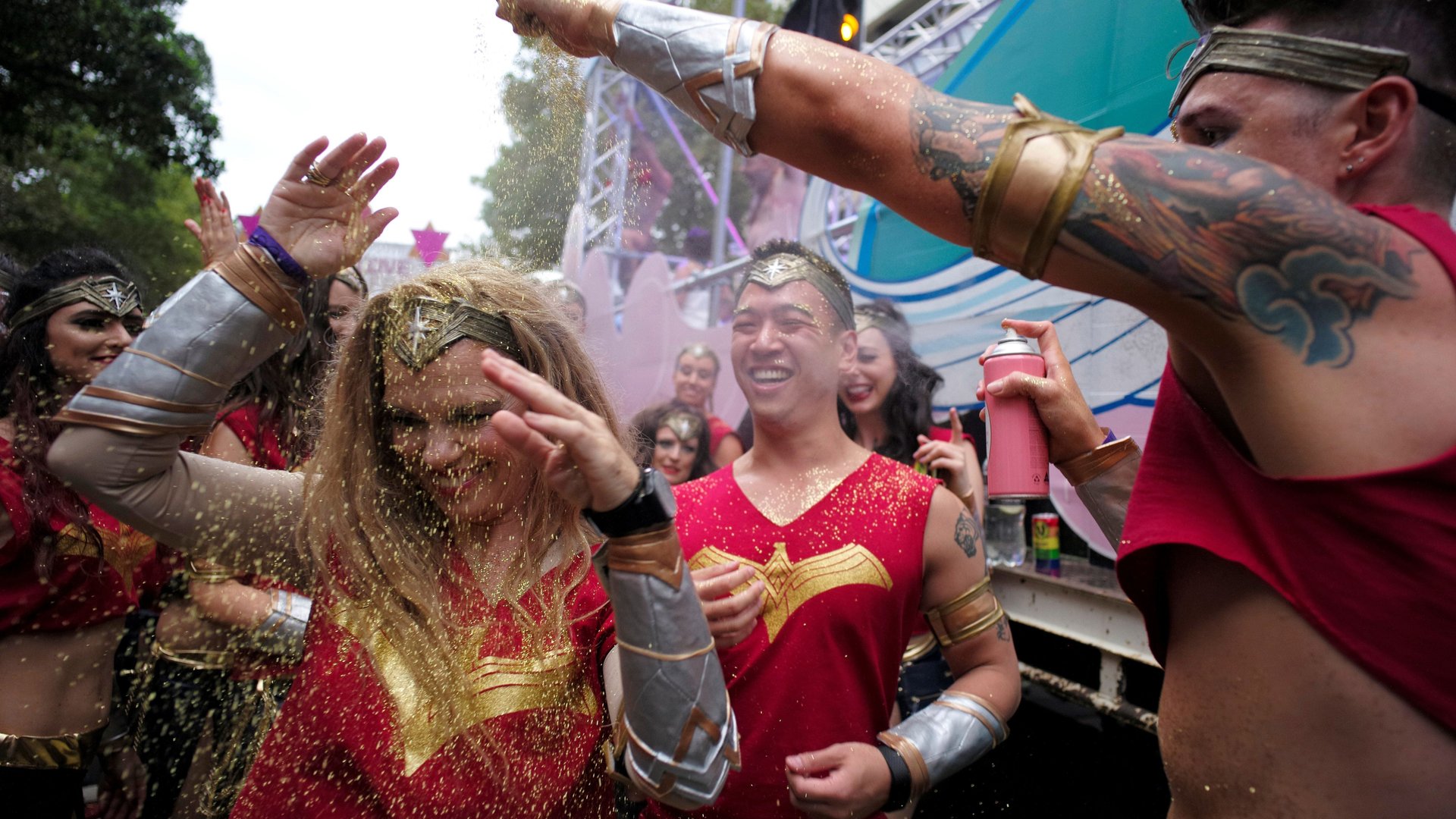
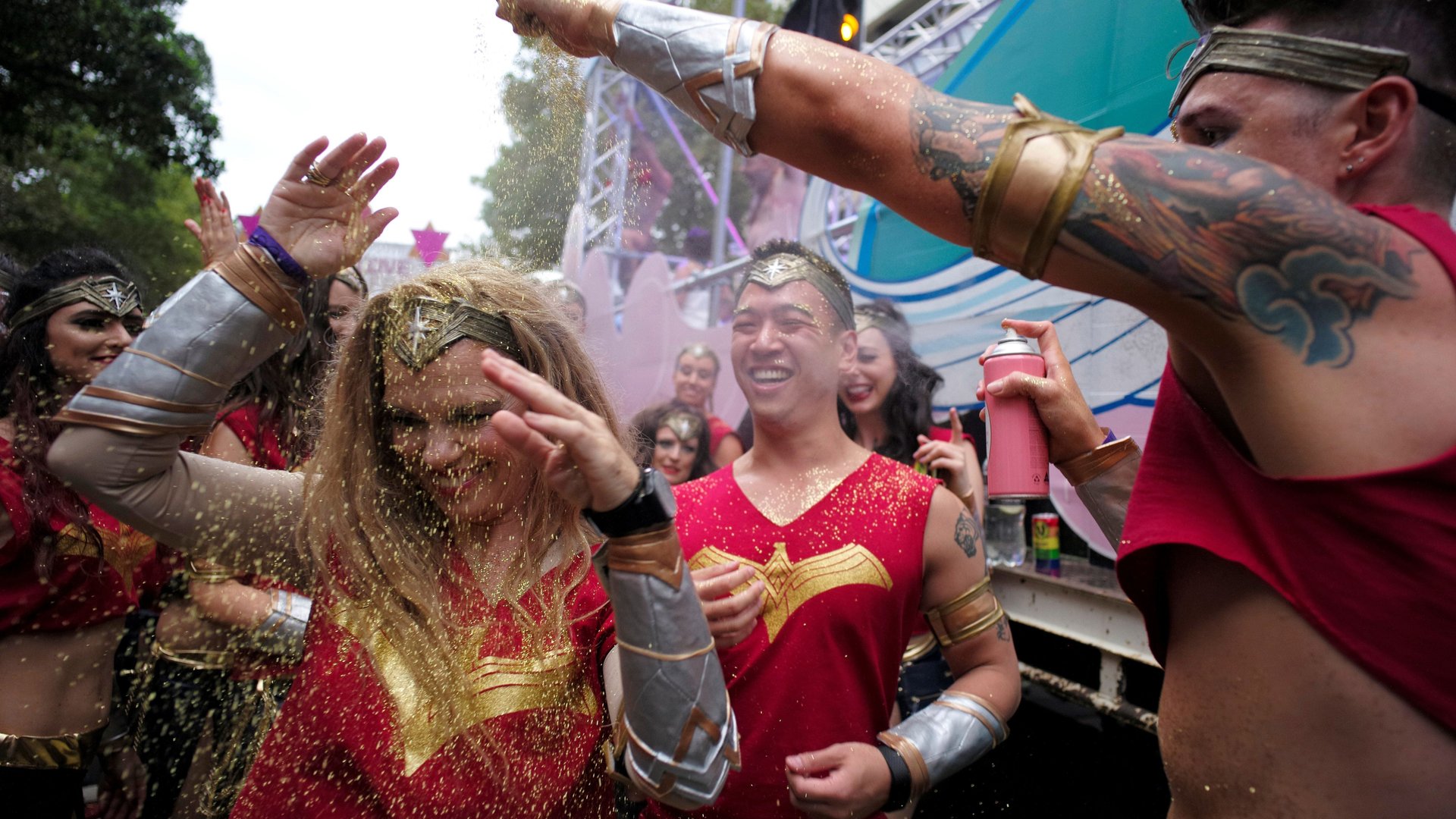
When I started my data analytics business several years ago, I quickly encountered what I now refer to as the “magic pixie dust” problem.
Pixie dust can manifest itself in many ways. It may be the business owner who is certain he or she is ready to invest in data and yet refuses to accept the price tag associated with it. The lawyer or financial advisor who has no problem charging $600 per hour for his or her time, but is mortified that a data expert commands comparable money. It is the smooth salesperson who promises you the world in a pitch meeting, but is not going to be on the other side of the computer doing the work and doesn’t understand at all what’s involved. It’s the mis-delivered promise sold to Hillary Clinton. In a presidential election won with margins so close that data should have made all the difference, it wasn’t to the benefit of Clinton, who spent millions on a widely criticized data program.
I co-opted the term “pixie dust” from a colleague and friend who is an alumnus of the Obama campaign. She uses it to refer to everyone wanting a piece of the seemingly magical electricity that was the Obama campaign and the belief it was fueled almost entirely by data and hence can be replicated to great success.
If only we could sprinkle some of that pixie dust, our own candidate would be saved!
The problem is, of course, that there was no pixie dust. While data was used in more ways than ever before, Obama was an exceptionally charismatic and good candidate who inspired the masses.
In a paper I wrote in 2010, I demonstrated that even the most robust Get Out the Vote campaigns cannot move the vote margin more than half a percentage point. The paper was buried before the ink dried on the page. Why? The promise of data fixing everything sells. The more accurate promise of hard work for marginal gains is a wet blanket unlikely to inspire the needed funding.
The same thing happens with the businesses I work with today.
Data can and will make the difference in an ecommerce algorithm where 0.1% lift could add millions of dollars in revenue. This is the promise of data, and yet a huge percentage of small and medium-sized business owners have been sold big, bold (and often inaccurate) claims that data will fix all that is wrong and outdated in their company.
Here’s how to tell when you are being sold vaporware.
Start by not being afraid to ask questions—lots and lots of questions
If you are shamed or meant to feel like you couldn’t possibly understand, move on. A good data scientist can explain to you in plain words what he or she will be providing you. Anyone who claims it’s too complex is hiding something.
Deep learning, a specific kind of machine learning, does have a natural black box approach, but the large majority of businesses do not have enough data to justify such an approach, and those who do should be beyond the point where they need someone to explain it to them.
Even if your business would truly benefit from a less transparent final product, there is no reason you cannot request a slimmed-down exploratory model be created. Exploratory models help everyone better understand the inner workings and they are usually a good indicator of potential problems as well as interesting patterns and trends.
Not sure where to start? Here are a handful of generic questions you can tailor to your organization:
- What can I expect as an end product, and how will it interact with my existing products and services? You should find out before you spend the money how you will deploy and integrate the product in your existing business. You don’t want to pay for analytics you can never deploy.
- How can we be sure this will work in practice? It does you no good to have a theoretically good model that fails in use. Always include a testing and evaluation component.
- What are the concerns/areas of consideration for a project like this? There are always challenges, you want to make sure you are hiring people who have a good understanding of what those challenges could be and how they will handle them.
- How can we avoid bias and feedback loops? This is a major issue in machine learning. Have a plan in place for both exploitation and exploration as well as bias testing or be prepared to see results tank over time or even be liable for discrimination.
There is more than one type of subject matter expert. Utilize them all
It is not in anyone’s best interest to have analysts who sit in ivory towers creating models without ever stepping foot in the field. If you are looking to bring analytics or data science to your business, then you are the subject matter expert of your business.
A good data scientist will spend hours interviewing subject matter experts such as you, your staff, and your vendors to make sure they understand all the implications and concerns of the real-life aspects of the business before he or she starts crunching numbers. Help your analytics experts understand the parts of the business you uniquely understand. Just don’t insist on specific solutions. That’s where you will want to use their expertise.
Beware of spurious correlations
One of the most interesting and dangerous promises of big data is the possibility of finding totally unknown and previously unpredicted patterns. This capability has led to many amazing discoveries, but has also lead to meaningless findings such as the correlation between the S&P 500 and butter production in Bangladesh. Make sure your practitioners are not hiding the goods under the hood so that you can spot these spurious correlations.
Do not discount the value of hard work
Often it’s as easy as trusting your gut. If it seems too good to be true, it probably is. Like any work, data work is hard work, otherwise everyone would do it. It requires specialized skills and years of experience to navigate and even still, is often subjective.
If you are presented a glorious picture of the unfettered promises of AI then you are probably being sold pixie dust. Resist the urge to buy it.
Navigating the promise and peril of a data-driven society can be intimidating for many people who are not in the business. Knowing it’s ok to ask questions and demand transparency should alleviate those fears a little. The right experts should help you to grow naturally in a way that does not disrupt your entire business (unless it’s not working and disrupting is your goal).
Just remember the words of Gordon B Hinckley: without hard work, nothing grows but weeds.
Talia Borodin is the founder of Amaro Science.