In 1908, the Guinness brewer William Gosset published a revolutionary paper titled “The Probable Error of the Mean.” Gosset, who published under the pseudonym “Student” at his employer’s request, often conducted experiments on the impact of new ingredients on the composition of his beer—such as the brew’s sugar levels.
Constrained by the fact that he was only able to collect a few samples to test, he would average the results. Still, he knew his best guess was probably not exactly right.
Gosset was determined to understand how close to accurate his average was likely to be, and how much that depended on the number of samples he had taken. His research into this issue led to his paper, the first major attempt to calculate the accuracy of estimates from different sample sizes. It included his t-table (pdf), which will be familiar to anyone who has taken an introductory statistics course.
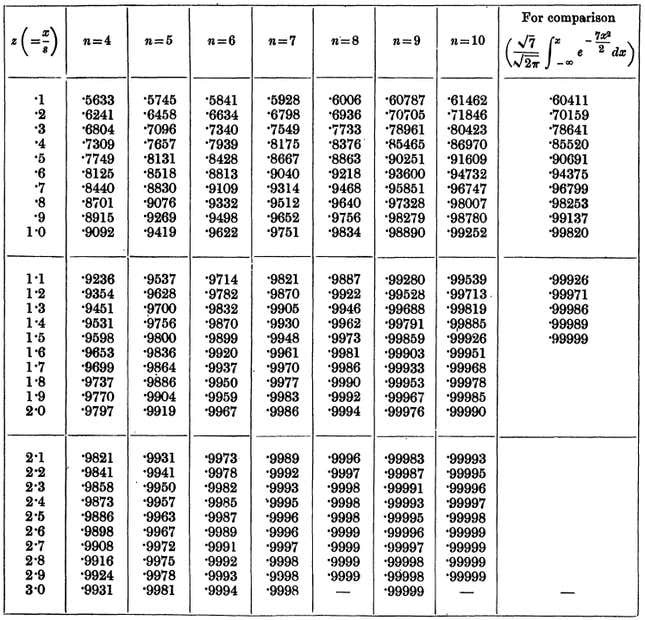
The genius and importance of Gosset’s research was not immediately heralded widely. But a few statisticians took notice. Among them was R.A. Fisher, a young man who would become perhaps the most influential statistician of the 20th Century. Fisher believed Gosset’s ideas could be used to test whether the difference between two groups was “statistically significant.”
In 1925, Fisher published Statistical Methods for Research Workers, the seminal book in which he explained the concept of statistical significance. Somewhat arbitrarily, Fisher chose to define statistical significance as a difference that had less than a .05 probability of occurring by random chance (in technical terms, this is called a p-value). A researcher who wanted to determine whether a new teaching method was effective might examine the test scores of a group of randomly chosen students educated in the new way against those who did not. If the scores of the students receiving the new method were so much better than those who didn’t that such a difference would only happen by chance 5% of the time, Fisher would say it was significant. (His logic proved to be flawed).
Fast forward more than a century later, and many researchers believe Fisher’s choice of .05 has led to a crisis in science. Many experimental findings in disciplines that use the .05 threshold—such as psychology, economics and medicine—turn out to be false. One large study, published in the journal Science, found that fewer than half of the findings published in three major psychology journals held up when replicated. Another study found that about 40% of experimental economics findings disappeared when the experiment was repeated. Though the .05 threshold is not entirely responsible for the problem—a lack of transparency in the research process is another factor—it is a primary culprit.
What’s to be done? A new proposal, authored by 72 prominent statisticians, economists, psychologists and medical researchers, offers a simple answer: Use .005 instead.
“This is an idea whose time has come,” the University of Southern California behavioral economist Daniel Benjamin, a lead author of the paper, told Quartz. “There is broad latent support for changing the language we use around statistical significance and tightening the standards.”
The exact proposal of the authors is that findings with p-values of between .05 and .005 would now be referred to as “suggestive” evidence, and the term “significant” would be reserved for findings that met the .005 threshold. Benjamin says that the solution, while not perfect, could do a lot of good in the short term. The paper presents research suggesting that it could reduce the number of false results in economics and psychology by half.
Benjamin admitted that choosing .005 was also a bit arbitrary, and that some of his coauthors argued for a lower number, but he says there is logic to it. A common interpretation of evidence falling below the .05 threshold is that this means there is a 95% chance that the finding is true. Benjamin says that using more sophisticated statistical techniques, this 95% chance is actually close to what a .005 p-value represents.
The goal of the paper is to win over two audiences. One, the authors hope academic-journal publishers will adopt their new statistical standards. Second, and more importantly, they hope researchers choose to adopt this language on their own. Benjamin points out that in two fields in which the p-value threshold was reduced, genetics and high energy physics, the change emerged from researchers who thought it was necessary for the maintaining the reliability of findings. His team hopes that medical researchers, economists and psychologists see that trust in their disciplines is now in peril—and a move from 0.05 to 0.005 might just be part of what saves it.