What a study of video games can tell us about being better decision makers
As summer was coming to a close, Elaine Rich and her team were poring over data on the pandemic, trying to determine how much the decision to close schools would help contain it. They were consulting epidemiologists and their own internal modeling, but the complexity of the question was still staggering. Just how important were schools to the virus’s spread?
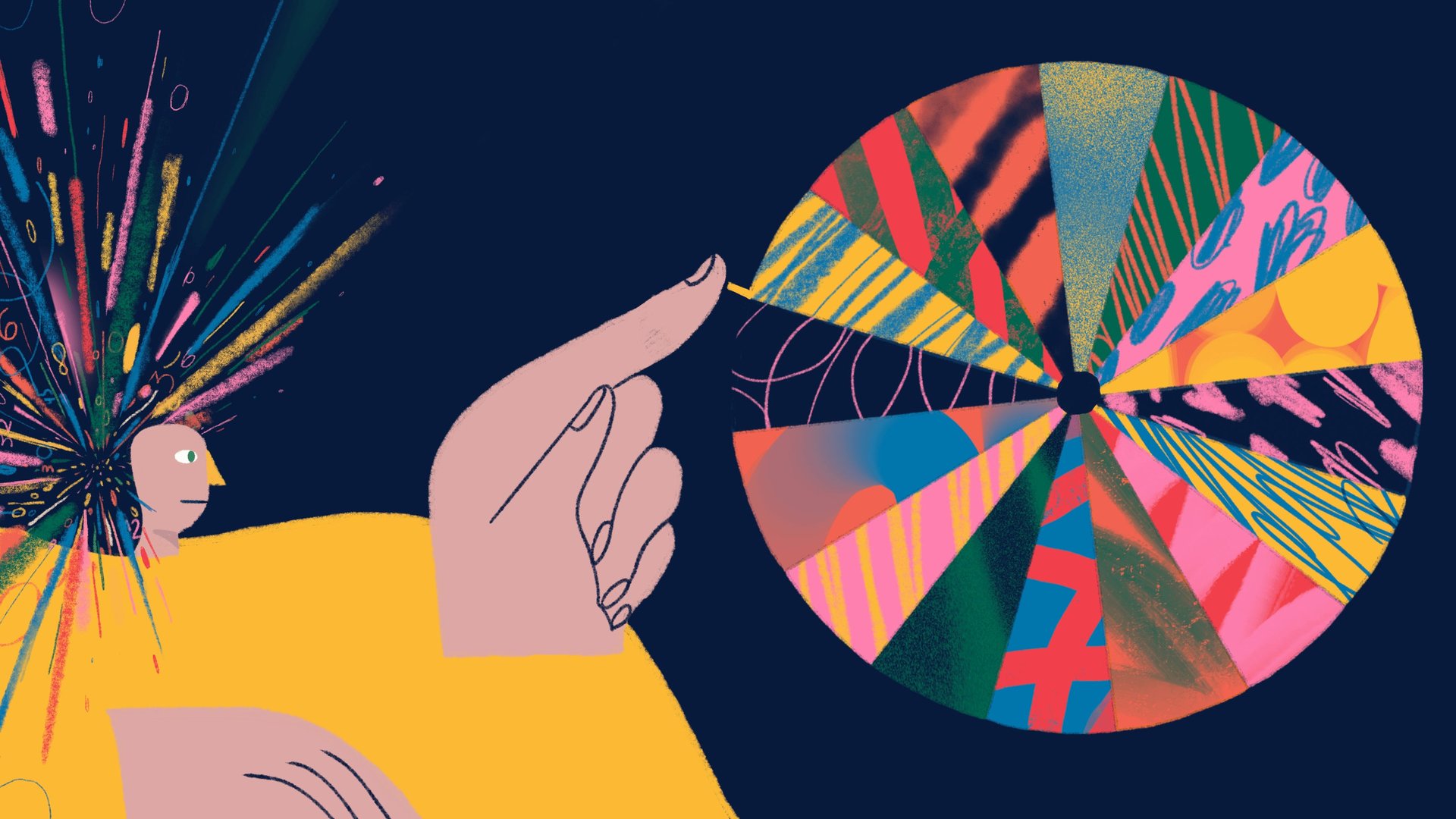
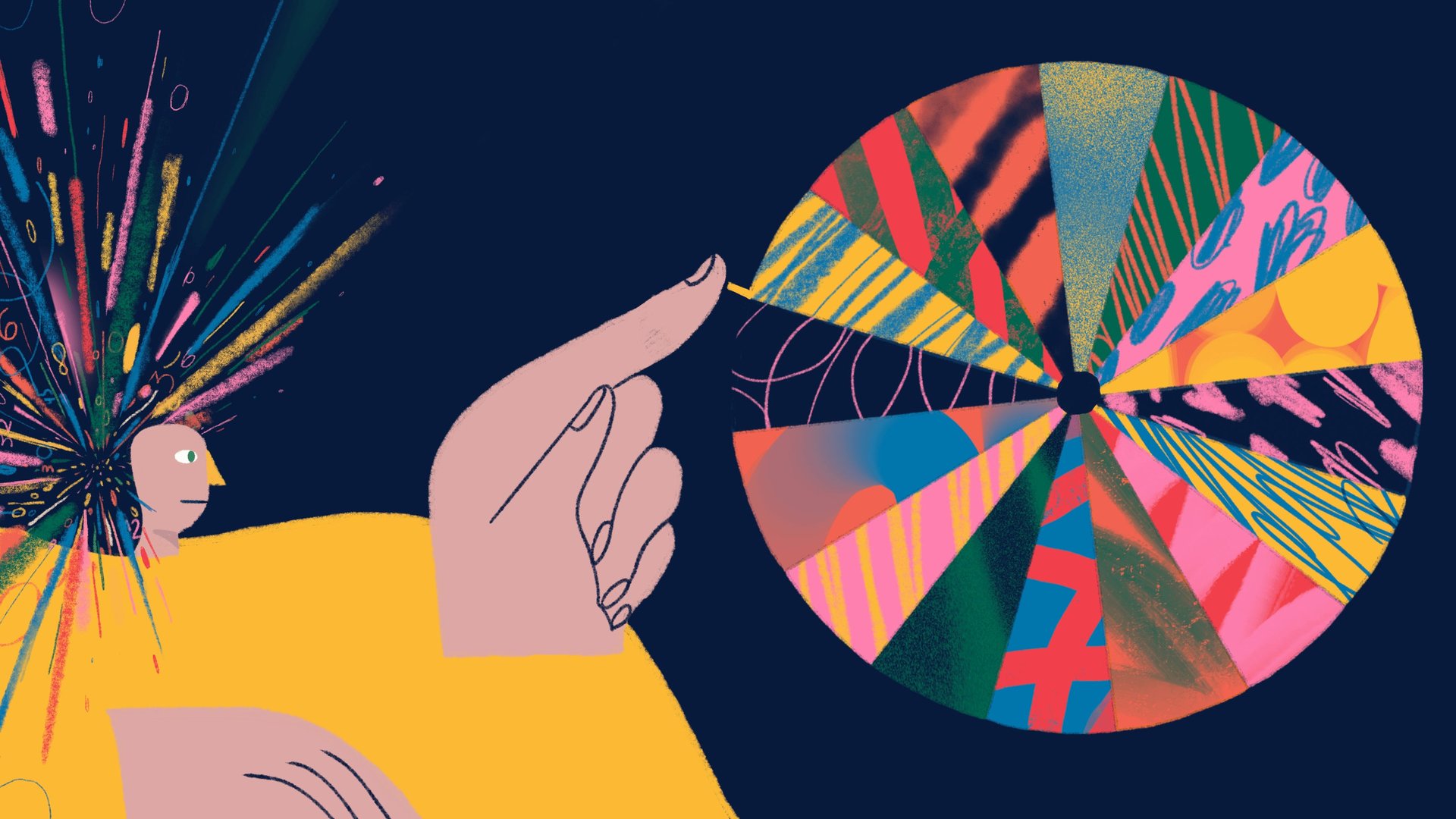
As summer was coming to a close, Elaine Rich and her team were poring over data on the pandemic, trying to determine how much the decision to close schools would help contain it. They were consulting epidemiologists and their own internal modeling, but the complexity of the question was still staggering. Just how important were schools to the virus’s spread?
The virus Rich and her team were studying was not Covid-19—in fact, it wasn’t real at all. It was a simulation conducted last year as part of a research project called FOCUS (Forecasting Counterfactuals in Uncontrolled Settings), sponsored by IARPA, the research branch of the US intelligence community. When the researchers chose an epidemiological simulation as one of the settings for their study, they didn’t know that the entire world would soon grapple with the very questions they were asking participants to answer.
FOCUS is the latest in a series of studies into how humans make forecasts—an essential part of making good decisions. Decision making is about formulating alternative plans of action, considering their consequences, and choosing between them. That middle step is all about forecasting. “Almost every decision we make depends on a prediction,” says Jenn Logg, an assistant professor of management at Georgetown University.
The lessons from IARPA-funded research on forecasting have informed the work of countless professionals—from intelligence analysts to software engineers to philanthropists—but they remain stubbornly underutilized in most organizations. Until Covid-19 cemented the stakes: Decision-making in uncertain situations can be literally a matter of life and death. Just as the novel coronavirus has caused a recognition of the need for pandemic preparedness, it has also illustrated the need for governments, companies, and individuals to reassess how they deal with uncertainty and how they make decisions.
Good decision-making requires imagining the outcomes of different potential choices, all but one of which will never actually occur. If a school district opens in September, the world never observes what would happen if that exact district hadn’t opened at that exact time. The road not taken is called the counterfactual, and there are whole branches of statistics devoted to how to measure it—even though it never actually occurs—including through randomized controlled experiments.
People and organizations rely on counterfactual thinking every day. What would sales have been last quarter if that top salesperson hadn’t quit? Would you have gotten the job if you weren’t late to the interview? Accurately imagining these counterfactuals is an essential part of decision-making, but we don’t know much about how people can get better at it. That’s what FOCUS set out to study using simulations and games, including a pandemic simulator and the popular computer game Civilization V.
Unlike in the real world, the FOCUS researchers knew what would happen to their fictional pandemic if schools were closed. They had run the simulation countless times, sometimes closing the schools and sometimes keeping them open. By comparing the data from those scenarios, they could calculate how big a difference school closures made, on average. To study how people estimate these counterfactuals with limited data, they pitted four teams of forecasters against each other, to see how well they could estimate different interventions’ impact on the pandemic’s spread.
Rich is a retired pharmacist living in Maryland, not an epidemiologist or policy wonk. In FOCUS, she was competing against students training to become intelligence analysts. But she is analytical, open-minded, and, like her teammates, she was selected based on her track record, from a previous research project, of accurately predicting world events. Her careful, critical, and probabilistic approach to thinking makes her an exceptional analyst. She is what psychologist Philip Tetlock, a professor at the University of Pennsylvania and one of the researchers working on FOCUS, calls a “superforecaster.”
Superforecasters are individuals who have proved themselves over several years of geopolitical forecasting tournaments, scoring in the top 2% for forecasting accuracy. The combined wisdom of the superforecasters has proven more accurate than that of professional intelligence analysts.
The story of the first round of FOCUS, told here for the first time, is about more than just a fascinating attempt to study how humans reason using video games. Together, the project, the participants, and the researchers involved can offer a primer on the science of forecasting and decision-making. Rich and her teammates have mastered a process for reasoning and deliberating that nearly every organization could benefit from.
“Our skills don’t guarantee that we’ll be good decision makers,” Rich said of her fellow superforecasters, “but not having our skills is a bad weakness—you need a little bit of what we’ve got.” With FOCUS, Rich and her teammates were asked to test the forecasting skills they’d honed predicting real-world events in a series of imaginary settings, including a simulated pandemic. They would not disappoint.

Table of contents
The Covid-19 counterfactual | Studying video games | Becoming a “superforecaster” | Making teamwork work | Why don’t companies take decision-making more seriously? | Predicting the pandemic

The Covid-19 counterfactual
On Feb. 8, 2020, state and city public health officials around the US began noticing a problem: The coronavirus test kits that the Centers for Disease Control and Prevention (CDC) had sent them were malfunctioning. “Taken by surprise, the CDC set out to remake the third component, a process that would take a week or more,” the Wall Street Journal reported. “The agency was confident it could fix the test, which worked in its own labs.”
That confidence was misplaced. By Feb. 19, the CDC still couldn’t get the third component to work; on Feb. 26, the US Food and Drug Administration advised public health labs around the country to use the original test, but without the third component.
How many more Americans would be alive today if the test had worked as planned from the start, or if officials had had less confidence in their ability to fix it and switched to an alternative sooner? There’s no way to know for sure because we can’t rerun history—these Covid-19 counterfactuals exist only in our minds.
As the pandemic rages on, every country is evaluating how it responded, to hold officials accountable and to strengthen public health systems for the future. Would the US have had more cases of Covid-19 than any other country if Donald Trump was not president? How many Swedes died because of the country’s early commitment to the theory of herd immunity? Would New Zealand have had as few cases as it has under different leadership? In every case, answering the question involves accurately imagining a counterfactual.
We use counterfactual reasoning all the time, usually without realizing it. Intelligence agencies like the CIA and MI6 do think a lot about it, because it’s an essential part of intelligence analysts’ jobs. If an analyst claims that Russia would be less aggressive today if NATO had not expanded eastward in the 1990s and 2000s, how can you vet their claim?
This is a fairly deep question, and one that comes up all the time whether we stop to think about it or not. If your head of sales says they would have had a good quarter except for the loss of a key salesperson, should you believe them?
The problem, again, is that you can’t rerun history: the CIA doesn’t get to see what would happen in the world where NATO doesn’t expand; you don’t get to know what sales would have been if that salesperson hadn’t quit; we don’t get to know the US case count if the CDC tests had worked from the start. In all three cases, there’s not enough data to answer the question with statistics and there’s no A/B test to run that can answer the question experimentally.
Enter IARPA and the FOCUS program. To study what makes someone good at thinking about counterfactuals, the intelligence community decided to study the ability to forecast the outcomes of simulations. A simulation is a computer program that can be run again and again, under different conditions: essentially, rerunning history. In a simulated world, the researchers could know the effect a particular decision or intervention would have. They would show teams of analysts the outcome of one run of the simulation and then ask them to predict what would have happened if some key variable had been changed.
But what sort of simulation could offer anywhere near the complexity of the real world—especially the messy world of geopolitics that intelligence analysts study? Among the simulations the FOCUS team chose to look at was the popular computer game Civilization V.

Studying video games
Civilization V is a strategy game about building empires. Players explore territory, build cities, fight wars, and discover new technologies. It takes hours to finish a game, and the range of possible decisions is endless—making it a good setting to test human analysts’ ability to think about counterfactuals. The game lets players play against each other or against the computer; for FOCUS, IARPA just let the computer play against itself. The challenge was to predict which of the civilizations would win the simulated game under different conditions.
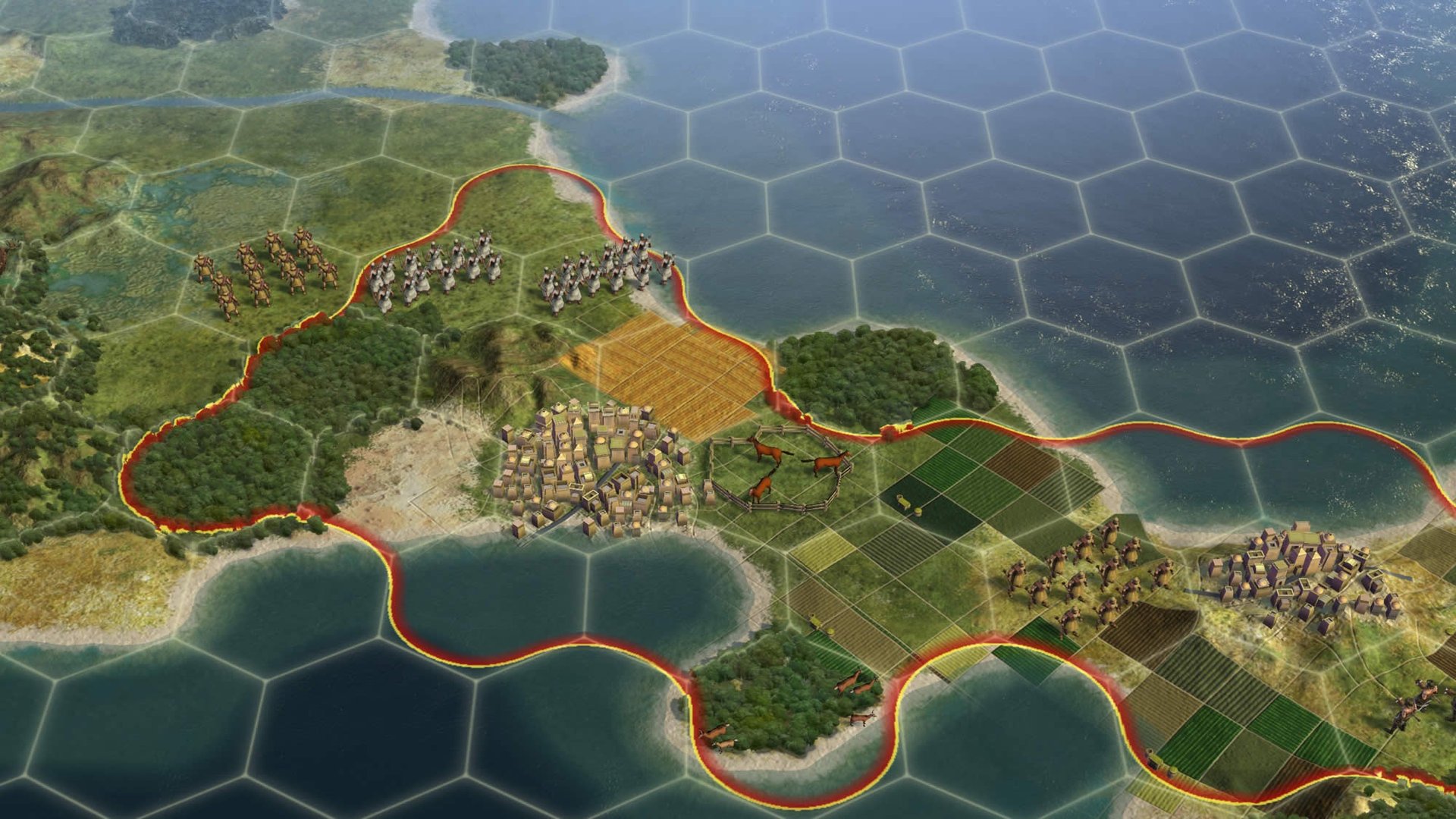
Rich had never played Civilization before FOCUS. Neither had her teammate Ezra Karger, a PhD student in economics and fellow superforecaster. They were allowed to simulate 50 games, so they had a bit of data on how things tended to go. And they had some Civilization players on their forecasting team. For each forecast, they were given a “World Report” outlining a game of Civilization V through turn 500. In one case, the world report showed that the Inca became the leading world power around turn 400 and by turn 500 were by far the most powerful empire in the world. (In Civilization, the players are named after real human societies but otherwise bear no resemblance to their namesakes.)
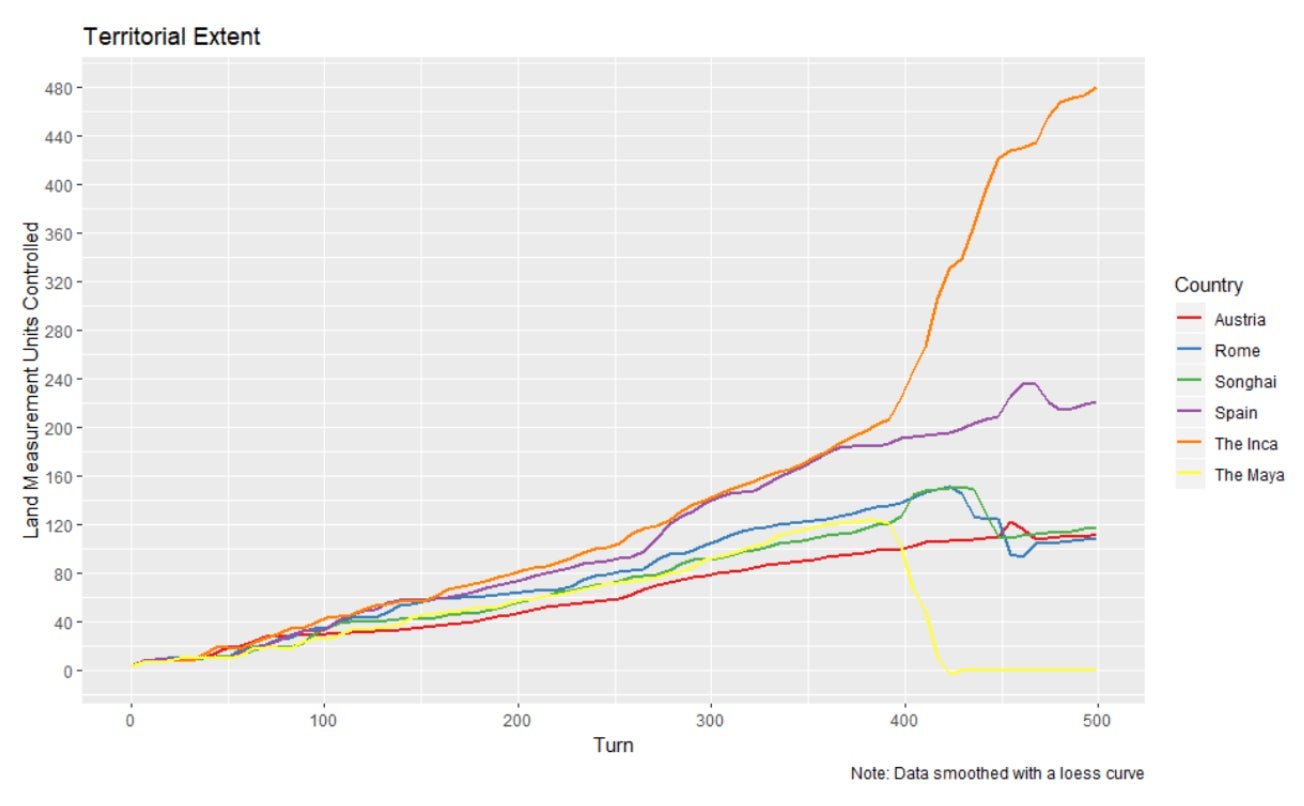
Armed with the report and their knowledge of the game, the forecasters were asked to answer the sort of “what-if” questions that have long annoyed historians and delighted authors of historical fiction. In this case: What if the river surrounding the Incas’ capital city had dried up starting on Turn 0? Would they still have become the most powerful society by turn 500?
Recall the point of this setup: If someone says Russia would be less aggressive if NATO hadn’t expanded, or that the US would have far fewer coronavirus cases if the CDC hadn’t botched the tests, it’s hard to know if they’re right because you can’t rerun history. By rerunning the Civilization simulation again and again, the researchers could measure how big an impact that river drying up would have on the Incas’ chances of success. And they could assess whether Rich and Karger were any good at estimating it.
Forecasting requires blending what the Nobel prize-winning psychologist Daniel Kahneman calls the “inside view” and the “outside view.” The inside view centers around the specifics of a given case, whereas the outside view asks how often different outcomes occur in general. Research on forecasting has found that most of us get too caught up in the inside view; we focus too much on the particulars and too little on calculating a baseline from a wider set of examples.
Here’s an example of the precise questions that FOCUS asked participants to answer:

Rich and Karger took different approaches to answering this what-if, both of which started with the outside view.
Before he even read the report, Karger looked at the data from the 50 simulations his team had been allowed to run, and calculated how often the population of civilizations at turn 150 fell into the various buckets. Fifty games with six players each meant 300 observations. By considering them all, Karger was starting with a baseline of how often different outcomes occurred.
Rich took a different approach. The World Reports that the forecasters were given were written by Johns Hopkins Applied Physics Laboratory, which does research on simulations. The report for this particular question was 57 pages of charts, tables, and analysis detailing 500 turns of Civilization play. Rich went straight to the report and did what she and her teammates call “gisting”—summarizing the 57 pages into about 200 words, describing the key aspects of the game while leaving everything else out.
World Report via IARPA
The trick with gisting is deciding what’s relevant and what’s not. Here’s an example of the kind of “gists” the superforecasters produced, this one written by a member of the University of Pennsylvania research team:
“The history of world 125 can be divided into three periods. Period 1 (approx. turns 0-300) is characterized by isolated and peaceful relations among civilizations exploring and expanding control over continents 1 and 3. In period two (approximately 300-400) the Incas and Spain emerge, respectively, as dominant over the Songhai and Maya in continent 1, and over Rome and Austria in continent 3, and use naval resources to compete for control of continent 2. In period 3 (approximately 400-499) the Incas and Spain compete with each other for survival or world domination by enormous investments and wars against each of their continental neighbors. In tumultuous period 3, the Incas match the historically greater resources of Spain by destroying the Mayans and appropriating their wealth and by deception and well-timed war against the Songhai. While Spain remains the dominant power on continent 3, Austria and Rome survive as coherent civilizations accepting their client status relative to Spain. The Inca dominate continent 1 and divide control with Spain of continent 2. The Songhai survive but they face a dismal future as the Inca are poised to do to them what was done to the Maya. World 125 at turn 499 features a bipolar cold war between the Incas and Spain.”
Gisting is a more qualitative way of taking the outside view. It’s an attempt to highlight the key factors that will affect a forecast and to leave the unimportant details aside.
Only with that work done did Rich and Karger turn to the crux of the question: How big of a difference would it make if the river dried up? To answer that, they scoured online forums about Civilization, quizzed their teammates who’d played the game, and debated the question on Zoom calls. They considered counterintuitive arguments like the possibility that it could be an advantage because, if the Inca were less powerful early on, other civilizations might focus their attacks on bigger threats.
Over the course of a year, 30 superforecasters answered 100 questions like this across four different simulations. They predicted the rise and fall of empires in Civilization, the spread of species in a simulation called CritterWorld, the winners of a game called ReconBlindChess, and the spread of a deadly pandemic in a simulator known to them as PurplePox.
In all these environments, the key to the superforecasters’ success was their ability to combine the qualitative with the quantitative and the inside view with the outside view. It’s a skill they’ve honed over years of practice.

Becoming a “superforecaster”
Rich got interested in forecasting through reading blogs like FiveThirtyEight, run by statistician Nate Silver. In 2011, through a link from the economics blog Marginal Revolution, she came across something called the Good Judgment Project, which was looking for volunteers to participate in a geopolitical forecasting tournament and research project. Good Judgment was led by Tetlock, the University of Pennsylvania psychologist, whose earlier research on forecasting had found that experts were often no better at predicting world events than random chance. In Good Judgment, Tetlock and his colleagues hoped to determine what made some people succeed in forecasting and to test whether the wisdom of the crowd could outperform professional analysts.
In her first two years of Good Judgment, Rich was asked to submit forecasts about various world events on her own. She’d submit estimates of how likely something was to happen in the form of a percentage—for example, the chance that there would be regime change in a particular country or that the price of oil would exceed a certain threshold. She declined to offer a rationale for her beliefs to the other forecasters, preferring to stay anonymous. “That was really important to me, because I didn’t have any credentials and I didn’t really want anybody to see me,” she said.
The Good Judgment Project assessed the accuracy of forecasts using what’s called a Brier Score, which is the squared difference between the forecast (stated as a probability) and the actual result. Lower is better. If you say something has a 90% chance of happening and it does, you’ve done well and will have a low Brier score, since the difference between 90% (your forecast) and 100% (the thing actually happened) is small. If you predict something has a 90% chance of occurring and it doesn’t, your Brier score will be high (bad) because the difference between 90% (your forecast) and 0% (it didn’t happen) is large.
When Brier scores are calculated across hundreds of forecasts, they can be reliable measures of a forecaster’s accuracy. By this and other metrics, the combined forecasts of the Good Judgment participants outperformed both a team of intelligence analysts and all of the other teams competing in a forecasting competition sponsored by IARPA.
But Tetlock and his colleagues weren’t content leaning purely on the wisdom of the crowd. They could see in the data that some of their amateurs were far better than others. They wanted to know if those high-performers would get even better if they worked together on a team.
The top performers in the Good Judgment Project tended to exhibit a certain style of thinking. They were highly numerate and knew to consider the “outside view.” They were open-minded and always looking for evidence that would contradict their view. They broke big questions down into smaller ones. And they tended to balance several competing theories against each other rather than rely on one explanation of how things would play out.
Rich exhibited these qualities. “I’m a generalist,” she said. “I don’t have a special understanding of history or world politics or anything like that, but I’m willing to look at things from different perspectives and read a lot of people.” She was also good at math and comfortable with probability.
After two years she was selected to join a team of top forecasters within Good Judgment, based on the accuracy of her predictions. Doing so meant dropping the anonymity and having to justify her forecasts to teammates.
“At least half the team had fancy credentials,” says Rich, “and I was like, ‘OK I’m not going to let my profession down.’” But Rich wasn’t just representing pharmacists. She was also the only woman on her 12-person forecasting team. “It wasn’t pleasant,” she said. “People were nice enough to me, but the combination of being a woman and being a pharmacist didn’t feel good. I don’t blame anybody for that but that’s just the way it was. It was awkward.”
Rich and the others did perform better when they were allowed to work together, according to research by Tetlock and his colleague Barb Mellers, also of the University of Pennsylvania. The superforecasters weren’t just individually strong at prediction; they also understood something important about working in teams.

Making teamwork work
Rich, Karger, and the Good Judgment team were only one of the participants in FOCUS. The project pitted four teams against each other. The “control group” in the experiment was made up of undergraduate and graduate students in the intelligence studies program at Mercyhurst University in Pennsylvania. The program trains students for careers in intelligence analysis, both in government and the private sector, and the students worked in small teams but were not given any additional training in counterfactual forecasting beyond what they’d learned in their studies. The other two teams came from Charles River Analytics and Raytheon BBN, both R&D firms.
IARPA’s aim in funding this research is “Can this be put in a training module in Quantico?” says Kaitlyn Coffee, project manager for the Good Judgment FOCUS team. IARPA put out a request for proposals, looking for researchers and teams who would help them learn what they hoped to from FOCUS. Raytheon’s pitch to IARPA was simple: “We’re not going to put together a team of experts. We’re not going to put together teams of professional analysts,” Alice Leung, one of the researchers, recounted. “We’re going to put together teams that are drawn from the general public.”
Unlike the Good Judgment team, Raytheon BBN couldn’t bank on the track record of its participants. They would have to design a process for accurate forecasting that anyone could use. “It wouldn’t be just training for geniuses or special tools that you needed to be an expert to use,” said Leung. In other words, it could be taught to a wide range of analysts across the US government.
Raytheon BBN put up ads in Dayton, Ohio, where a Raytheon subcontractor has an office, asking for volunteers willing to spend 40 hours over two weeks. They didn’t take just anyone. Since previous research had shown that intelligence, familiarity with probability, and open-mindedness all correlate with accuracy, they administered intelligence and personality tests and set a performance cutoff for participating.
The key to their approach was a teamwork best practice that many teams still ignore: a structured balance between individual and group deliberation.
“If you have people work in teams for brainstorming, sometimes you get premature consensus,” says Leung. “You get group think. We knew we wanted people to do some individual work, and then talk with teammates, and then do some more individual work, and then talk to teammates.”
The superforecasters do something very similar, based on a forecasting process called the Delphi Method. In Delphi, which was invented by the RAND Corporation during the Cold War, every participant first makes a forecast on their own and provides their reasoning, anonymously. Then everyone reads each others’ forecasts and reasoning and makes a second forecast based on that information. In some versions, participants talk in between the estimates to explore each others’ reasoning in more detail. It’s one of the first things Tetlock and many other decision experts recommend groups try to improve their forecasts.
The Raytheon BBN team adopted a Delphi-like approach of alternating individual and group work and extended it across the entire forecasting process. Individuals were tasked with taking the “outside view,” then came together to discuss how they arrived at their conclusions. Then they went off and refined that view on their own, by narrowing in on similar cases and thinking about the causal forces in play. Then they came together to discuss as a group. And so on through an 8-step process.

Why don’t companies take decision-making more seriously?
The process that Raytheon BBN designed for its amateur forecasters was based on lessons from research that most teams and organizations still ignore. The most common way that most teams make decisions is by coming together, talking informally for a bit, and then letting the person in charge decide. Many of those discussions are a complete waste of time.
In a recent study, Mellers, of UPenn, and her colleagues had participants estimate the answers to quantitative trivia questions, like the population of different countries, first individually and then through group discussion. After the discussions, participants separated and had the chance to update their answers. In nearly every case, the participants said the group discussion helped their accuracy. They became more confident afterward and their estimates became more similar. But only about half of the participants actually became more accurate as a result of the group discussions, and the group average improved only a little more than half of the time. In other words, more than 40% of the discussions didn’t help at all.
Mellers found that group discussion helped in cases where group members’ confidence was correlated with their actual knowledge. When the people who are most confident are also the ones who know the most, talking together improves the group’s overall accuracy. But when confidence and knowledge are negatively correlated—when the most confident people know the least—group discussion makes the group less accurate, because people treat confidence as a signal of knowledge and defer to their most ignorant members.
When a group’s process for working together improves accuracy, it’s called “process gain.” When the process makes the group less accurate, that’s “process loss” and processes that don’t change accuracy at all are “process neutral.” Although Mellers’ research shows that a two-step, Delphi-style process is no guarantee of process gain, it’s almost certainly better than the process most companies use, going straight to group deliberation without asking for independent judgments beforehand. Why don’t organizations invest more time searching for process gain?
“They miss the value of [good forecasting],” says Don Moore, a psychologist at Berkeley and author of Perfectly Confident: How to Calibrate Your Decisions Wisely. “They don’t consider the massive opportunity costs that they’re paying by not getting better at forecasting.”
Part of it is that informal, group discussion feels productive, says Logg of Georgetown. “People think sitting in a room and making a prediction is useful,” she says, despite the fact that starting with independent judgments and following a structured process is usually better.
Danny Hernandez, who has consulted with several companies on improving forecasting and is now a research scientist at OpenAI, a California-based research company, says the problem is the user experience. Training in forecasting or confidence calibration usually involves being shown that you know less than you think you do, so most people don’t enjoy it. For that reason, he says, “we’re in the extreme early adopter phase” of organizations learning from forecasting and decision research. (Quartz has created a tool for members to help practice overcoming overconfidence bias.)
If Hernandez is right, then there’s opportunity for software to help by making the experience easier or more fun. Apps for Slack and Microsoft Teams like Cloverpop and Polly make structured decision-making easier by interjecting simple polling into team conversations. Though neither offers a Delphi mode out of the box, Cloverpop is designed to encourage teams to go back and review past decisions in order to improve.
It’s worth considering two other explanations as to why organizations don’t adopt forecasting best practices. The first is that decision-making is about much more than prediction and forecasting, which is true. But forecasting is one of the toughest and most central aspects of good decision-making. “Every decision depends on a forecast of its consequences,” says Moore. And so “every organization needs to make forecasts.”
The second objection is that the lessons from the Good Judgment Project don’t generalize. Sure, maybe the temperament, habits, and process the superforecasters used to predict world events worked in that specific context, but there’s no guarantee that they will work in every industry or profession.
FOCUS was a test of how far those skills would generalize. Would being good at predicting geopolitics translate into predicting interventions in games like Civilization 5?
The FOCUS project has only completed one round and no research has yet been published documenting the results. But so far, the answer seems to be yes. The Good Judgment team placed first in Round 1, with a team accuracy score 0.6 standard deviations above the control group. They won three out of five rounds of Civilization V and every round of the other simulations. (Raytheon BBN placed second, 0.37 standard deviations above the control, and Charles River was comparable to the control group.)
“FOCUS was the third major research project in a row where the superforecasters walloped the competition,” says Warren Hatch, CEO of Good Judgment Corp., a firm that sells the superforecasters’ predictions and methods to clients. “Whenever we’ve had an opportunity to benchmark the superforecasters… they’ve come up tops.”
Moore, who was involved in the Good Judgment Project but not in FOCUS, remains more skeptical that the lessons from the superforecasters can generalize to other settings. His own research has suggested that overcoming overconfidence in one domain is no guarantee of overcoming it in another, and he thinks something similar could be true of forecasting. “My wife and family will still tell you that I am overconfident in all sorts of ways,” he said.
But while most organizations haven’t adopted the lessons from forecasting research, some corners of the economy are starting to take an interest.
“There’s a lot of receptivity in finance,” says Tetlock. “The big actors in Wall Street are under no illusions. They know they’re in the forecasting business.” Silicon Valley has been receptive as well, he said. The intelligence community has long been interested, as evidenced by its decades-long funding of research. But Covid-19 has led other government agencies to take an urgent interest, too.

Predicting the pandemic
Of all the simulations used in FOCUS, PurplePox, the pandemic simulator, was the hardest to predict. “My main takeaway from that is it’s very hard to predict pandemics,” Karger told Quartz. “[It’s] really hard to predict exponential growth.”
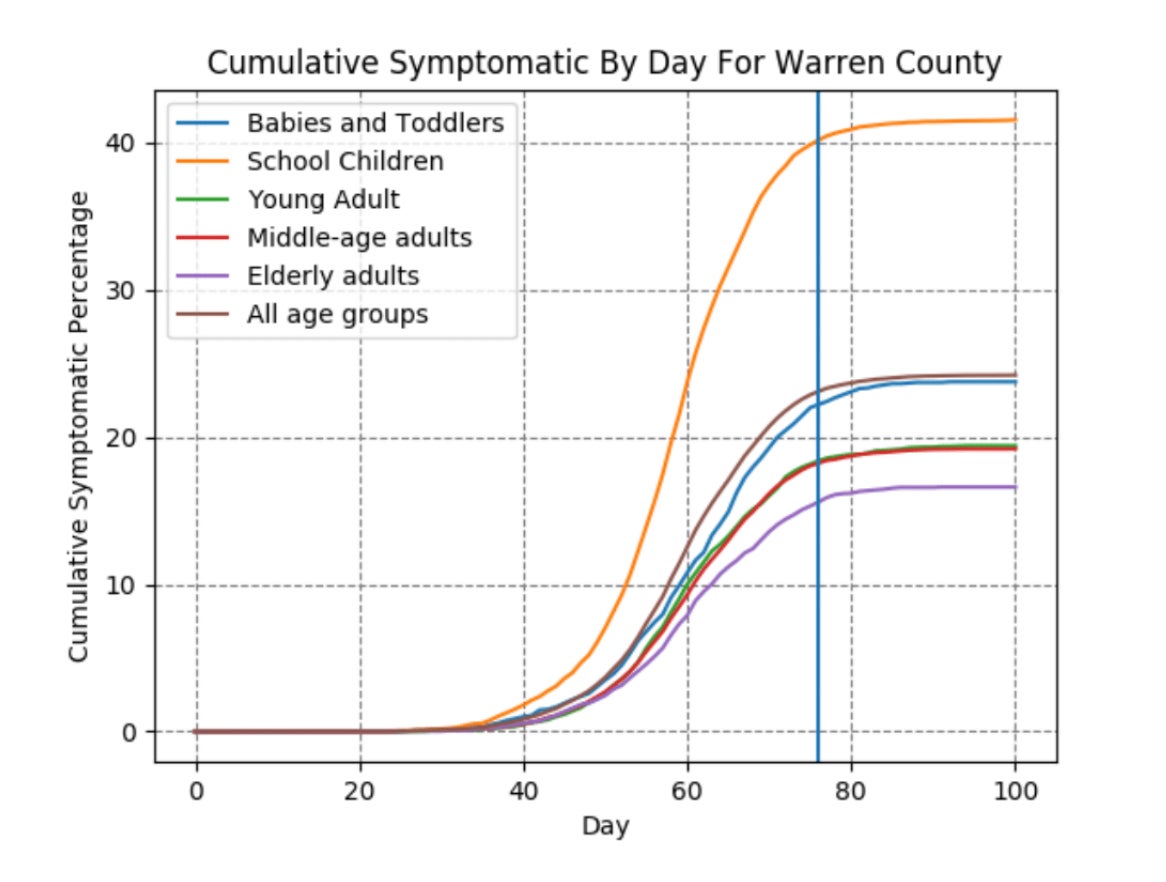
Predicting the course of the PurplePox pandemic was particularly hard because the forecasters were given data for just one county on a fictional island and were asked to predict what would have happened across the entire island if schools had closed early on. (This setup was not unlike epidemiologists trying to predict the course of the US pandemic by looking at what had happened in Wuhan or Italy.)
Rich and Karger took the same kinds of approach as with Civilization. Karger read up on the models epidemiologists use to predict pandemics and trained one using the data they’d been given. Rich produced a 150-word “gist.”
They also had a chance to talk to epidemiologists, which both Rich and Karger said helped a lot. Their team was the most accurate across all of the pandemic predictions in FOCUS.
Tetlock and Mellers asked the epidemiologists who had volunteered to be a resource for the superforecasters to make their own predictions. But the experts were “way off,” Mellers said, despite their familiarity with the subject matter and the type of models on which the PurplePox simulation was based.
The superforecasters were better at predicting the simulated pandemic than trained epidemiologists, but what about predictions in the real world? There, too, there is evidence that experienced forecasters are able to outperform experts.
For six weeks starting in mid-April, the University of Massachusetts at Amherst asked infectious disease experts from Johns Hopkins, Harvard, the National Institutes of Health, and elsewhere to make predictions about the course of the Covid-19 pandemic. Good Judgment Corp. participated as well, posing the questions on their open platform, where anyone can forecast. The Good Judgment forecasters beat the experts five out of six weeks, according to data that Hatch provided to Quartz. He thinks the superforecasters would have done even better.
The difference, Hatch believes, is about process. Forecasters on the Good Judgment platform are “taking advantage of the best practices and the experts are not. The experts are still doing it the old-fashioned way. They will put in a lot of work to come up with their number, they’ll send it in, and that’s it.” For the Good Judgment forecasters, that’s just the first step, followed by pooling of information, sharing notes, and revising. “I would wager that if [the epidemiologists] took advantage of best practices, they would see a pretty rapid improvement in what they do.”
That is perhaps the biggest lesson of FOCUS: It pays to follow a more formal process when making predictions or important decisions. The superforecasters have lots of other things going for them—smarts, statistical fluency, open-mindedness—but most of all they are deliberate. Whether working alone or in groups, they follow a step-by-step process designed to help them be as accurate as possible. Most organizations could do much better simply by finding a version of that process that works for them.
This spring, the state of California took an interest in what the superforecasters were up to. Through Good Judgment Corp. the superforecasters are now working with the state government to forecast Covid-related questions so that California can make decisions based on the best available predictions. The company has also published a public dashboard of the superforecasters’ assessments of the pandemic, including the number of total cases and deaths that will be recorded by March 2021.
The most likely scenario, according to the superforecasters, is that a vaccine is less than seven months away.
Correction: An earlier version of this article incorrectly attributed a gist summary to Elaine Rich, instead of a member of the University of Pennsylvania research team. The last name of Alice Leung of Raytheon BBN was also originally misspelled. Raytheon BBN works with a subcontractor in Dayton, Ohio, not a subsidiary. The “superforecasters” scored in the top 2% of study participants, not the top 5% as originally stated.