How brand-new words are spreading across America
Words spread like weeds—seemingly at random but actually governed by invisible forces. Look away for too long, and suddenly new ones are emerging from who knows where.
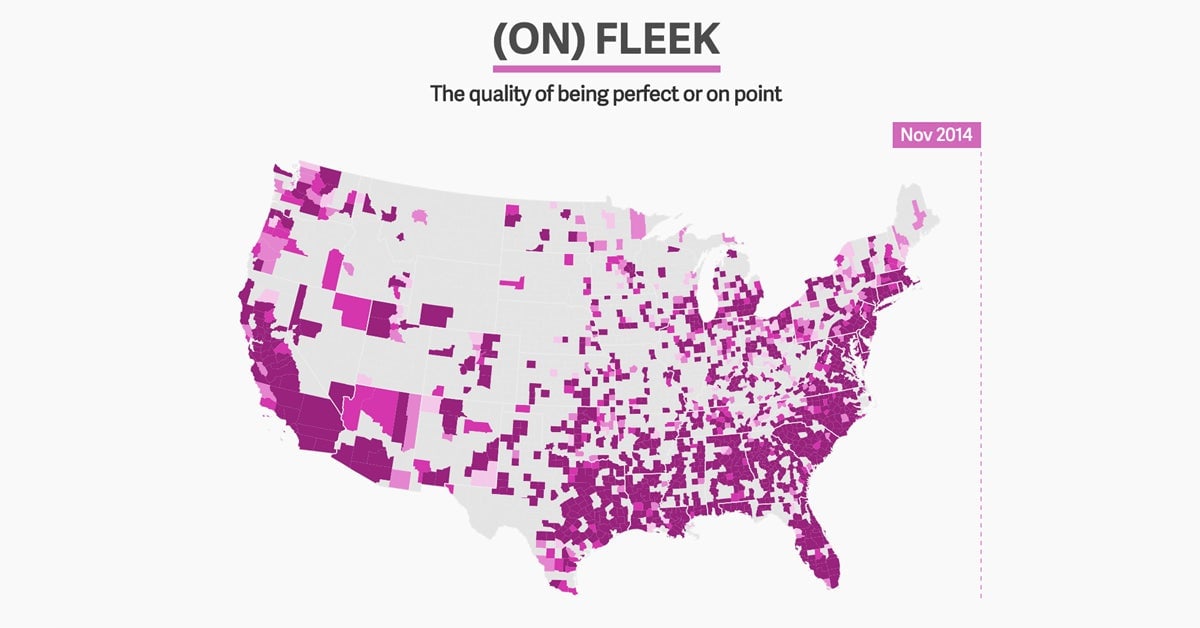
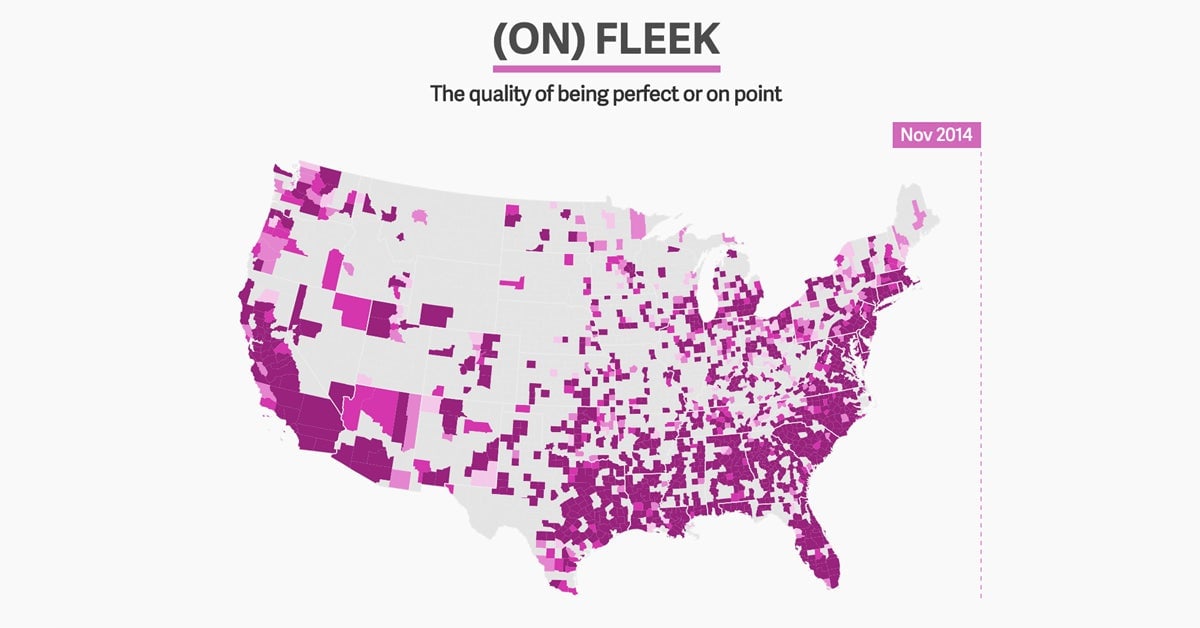
Words spread like weeds—seemingly at random but actually governed by invisible forces. Look away for too long, and suddenly new ones are emerging from who knows where.
The uncertain and gradual growth of words makes it nearly impossible to pinpoint where they started or how they caught on. But that is starting to change, as linguists draw on a wealth of data about word usage from social media services like Twitter.
Jack Grieve, a forensic linguist at Aston University in Birmingham, England, has been examining a dataset of nearly 9 billion tweeted words to identify the new American vocabulary. In a forthcoming study, he looked for words that were rarely used on Twitter in late 2013 but became common throughout 2014. Many of these “emerging words,” from baeless to xans, are included in the above graphic.
Identifying these words is interesting enough, but it doesn’t tell us how they came to be. Quartz worked with Grieve to visualize how these new words spread across the United States, using millions of tweets that had the user’s location attached to them. The maps reveal how common a word is in each county in a given week and how it spread over the course of the year.
“We know almost nothing about how new words spread, and this is a way of really seeing it,” Grieve said. “It’s pretty exciting to see real-time how words that are current are spreading out.”
You can see, for example, how on fleek exploded almost simultaneously across the country last year. The phrase, which roughly translates to perfect or on point, was a linguistic surprise hit. It didn’t start with a celebrity or brand trying to coin a new phrase. What set it off was Kayla Newman, a not-yet-famous Vine user, saying, “Finna get crunk. Eyebrows on fleek.”
From there it took off, fast. On fleek got picked up by IHOP, Taco Bell, and Kim Kardashian. Now it is fully in the lexicon, used regularly on Twitter as though it existed for many years, not just one. It is fundamentally a borderless word, native to the internet. The same is true of some other emerging words identified by Grieve, like amirite (“Am I right?”) and faved (to favorite a tweet).
That is not how most words spread. There is no clear inflection point, backed by bandwagoning celebrities, skyrocketing the word into wider usage. More commonly, words start in a particular region of the country and spread steadily from there. Fuckboy (loser) came out of coastal urban centers. GMFU (Got Me Fucked Up) was at first heavily concentrated in Louisiana.
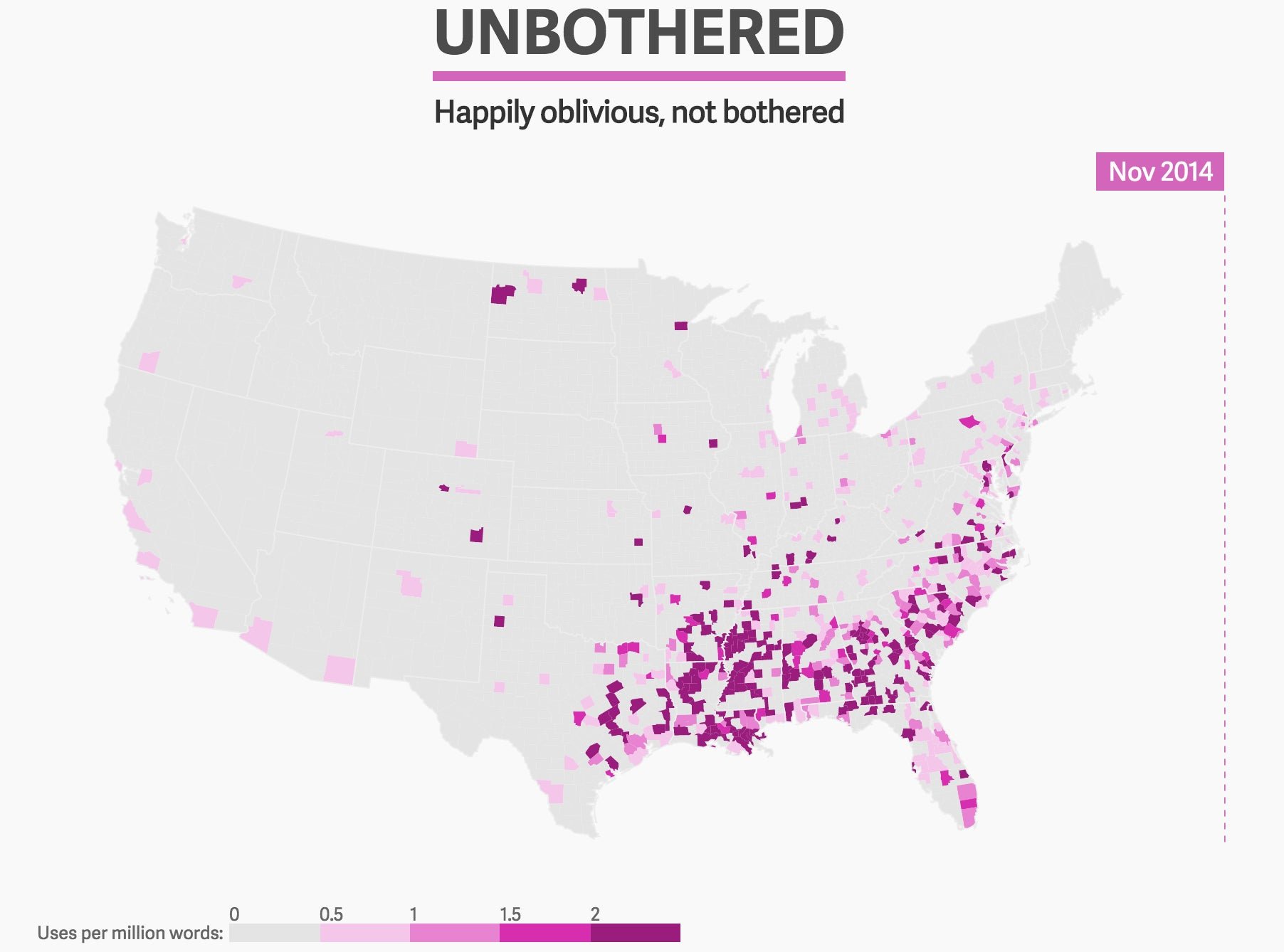
One region is particularly influential: the south. Several of Grieve’s emerging words got their start there. It’s true of boolin (chilling), baeless (single), bruuh (bro), unbothered (happily oblivious), to name a few.
This tells us two things. First, it is evidence of the “north-south split,” a linguistic divide separating two dialects of American English at the Mason-Dixon Line. Grieve called it the “strongest dialectical pattern in the United States.” Some of these fast-growing words, like unbothered, have barely left the south at all. Perhaps it will reach the north this year.
Second, we can see how African-American English is largely responsible for the coinages that secure a place in the lexicon. New words on Black Twitter grow to be used on the rest of Twitter. Some words—famo (friends and family) and tooka (marijuana)—appear particularly born of black communities in the south and certain northern cities like Chicago and Detroit.
The maps also show that successful new words can grow exponentially. As more people use a word, more people hear and learn it, leading more to use it, and so on. This is especially true of a phrase like on fleek, which spread like a meme:
Twitter offers an unprecedented dataset, or corpus, of language use in close to real-time. It probably over-indexes for certain words, but that is more of an asset than a liability in the case of this research: People using a platform like Twitter may also be early adopters of new words. In any event, tweets are a more natural representation of word choice than many other possible corpora.
“If you’re talking about everyday spoken language, Twitter is going to be closer than a news interview or a university lecture,” Grieve said.
The tweets he analyzed were collected by Diansheng Guo of the University of South Carolina. To identify emerging words, Grieve first sorted all words in his dataset by county and removed any that were used fewer than 1,000 times throughout the 9-billion word corpus. He then calculated the relative frequency of the remaining 67,000 words, identifying “rare” ones—those used fewer than once per million words. This helps remove some noise, but lower thresholds could reveal other fast-moving words.
Finally, to make sure the words could be considered new, Grieve threw out any found in another important dataset: the dictionary.