Reinforcement learning, a key Google DeepMind algorithm, could overhaul news recommendation engines and greatly improve users’ stickiness. After beating a Go Grandmaster, the algorithm could become the engine of choice for true personalization.
My interest for DeepMind goes back to its acquisition by Google, in Jan. 2014, for about half a billion dollars. Later in California, I had conversations with artificial intelligence and deep learning experts; they said Google had in fact captured about half of the world’s best A.I. minds, snatching several years of Stanford A.I. classes, and paying top dollar for talent. Acquiring London startup DeepMind was a key move in a strategy aimed at cornering the A.I. field. My interlocutors at Google and Stanford told me it could lead to major new iterations of the company, with A.I. percolating in every branch of Google (now Alphabet), from improving search to better YouTube recommendations, to more advanced projects such as predictive health care or automated transportation.
Demis Hassabis, DeepMind’s founder and CEO, a great communicator, gives captivating lectures—this Oxford University one is among his best, delivered on Feb. 24. The 40-year-old PhD in cognitive neuroscience, and a computer science graduate from MIT and Harvard, offers this explanation of his work: “The core of what we do focuses around what we call Reinforcement Learning. And that’s how we think about intelligence at DeepMind.”
[Hassabis then shows the following diagram]
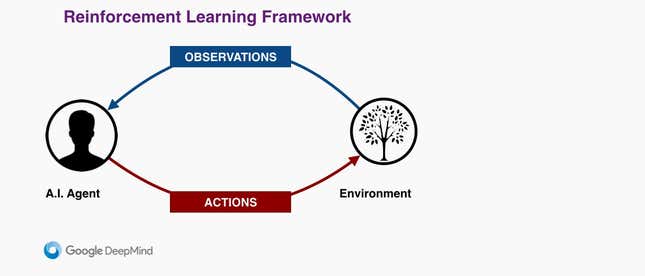
He continues:
We start with the agent system, the A.I. That agent finds itself in some kind of environment, trying to achieve a goal. In a real-world environment, the agent could be a robot or, in a virtual environment, an avatar.
The agent interacts with the environment in two ways. Firstly against observations through its sensory operators. We currently use vision but we start to think about other modalities.
One of the jobs of the agent system is to build the best possible model of the environment out there, just based on these incomplete and noisy observations that [the agent] is receiving in real time. And it keeps updating its model in the face of new evidences.
Once it built this model, the second job of the agent is to make predictions of what is going to happen next. If you can make predictions, you can start planning about what to do. So if you try to achieve a goal, the agent will have a set of actions available. The decision making problem is to pick which action would be the best to take toward your goal.
Once the agent has decided that based on its model, and its planned trajectories, it executes actions that may or may not make some changes in the environment, and that drives the observations…
Reinforcement learning is a highly complex process. First, the observed environment is very noisy, incomplete, and largely consists of unstructured pieces of data. When DeepMind decided to tackle basic Atari games like Breakout and Pong, the input was nothing but raw pixels, and the output was predictions—likely target position—and then actions—racket placement. All of the above aimed at maximizing survival and score. After a few hundreds games, the machine was able to devise—on its own—creative strategies that would surprise even its creators (read here or view this video, time code 10:27).
Over time, the tests will migrate to more complex environment such as 3D games in which it becomes harder to distinguish the pattern of a wall from a useful piece of information.
DeepMind’s future goals involves dealing with very large and complex sets of data such as genomics, climate, energy, or macroeconomics.
Regardless of the nature of the input stream, the principle is roughly the same. The A.I. system relies on a deep neural network to filter raw sensory data and form meaningful patterns to be analyzed. It then builds an optimized statistical model, updates it in real time, and derives the best possible actions from the set of observations available at a given moment. Then the whole system loops back.
How does this connect to improving news production?
Before we get into this, let’s roll back a little bit.
For a news production system, recommending related stories or videos is the most efficient way to increase reader engagement. For media who rely on advertising sold on CPM or a per-click basis, raising the number of page views per reader has a direct impact on ad revenue. Paid-for media are less sensitive to page views, but reminding readers of the breadth and depth of an editorial production is a key contributor to a news brand’s status—and a way to underline its economic value.
But there is a problem: today’s news recommendation systems are often terrible.
To display related stories or videos, publishers unwilling to invest in smart, fine-tuned systems have settled for engines based on crude semantic analysis of content. Hence embarrassing situations arise. For example, a piece about a major pedophile cover-up by the French clergy will trigger suggestions about child care. Or another where an intellectual debate will trigger a suggestion for a piece on spelling or waste management. The worst are stories automatically proposed by Outbrain or Taboola and picked up all over the web: not only are they the same everywhere, but they tap into the same endless field of click-bait items. The only virtue of these two systems is the direct cash-back for the publisher.
Something needs to be done to improve recommendation systems. A.I. and reinforcement learning offer a promising path.
In Demis Hassabis’ demonstration, the important words are: environment, observations, models, predictions, and actions. Let’s consider these keywords in the context of news production and consumption.
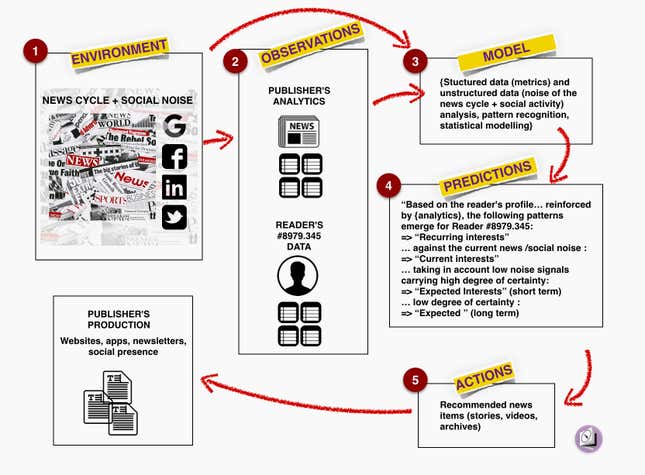
Environment
The environment is dual. The external side is built on the general news cycle. At any given moment, automatically assessing a topic’s weight is reasonably easy, but even though they’re critical to predicting how the news cycle will evolve, detecting low-noise signals is much trickier. As for the internal news environment, it is simply the output of various contents produced (or curated) by the newsroom.
Observations
Observations are multiple: they include the vast range of available analytics, again at two levels: how a piece of contents is faring in general (against the background noise or the competition), and how each individual reader behaves. Here, things become interesting.
Models
Models are then fed with a mix of statistical and behavioral data such as: “Stories [x] containing [semantic footprint] perform well against context [of this type of information].” Or: Reader #453.09809 is currently interested by [topics], but she has on her radar this [low-noise topic] that is small but growing.
Predictions
Predictions detect both contents and topics that have the best lift in the news cycle and pique the reader’s interest, dynamically, in real time.
Actions
Actions will then range form—putting stories or videos in front of the audience, specifically at the individual level. Personalization will shift from passive (the system serves stories based of the presumed and generally static reader profile) to dynamic (based on current and predicted interest).
Demis Hassabis makes clear that enhanced personalization is on DeepMind’s roadmap: “Personalization doesn’t work very well. It currently sums up to averaging the crowd as opposed to adapting to the human individual.”
It would be unrealistic to see a news outlet developing such an A.I.-based recommendation engine on its own, but we could easily foresee companies already working on A.I. selling it as SaaS (Software as a Service).
A new generation of powerful recommendation engines could greatly benefit the news industry. It would ensure much higher reader loyalty, reinforce brand trust (it recommends stories that are both good and relevant), and help build deeper and more consistent news packages while giving a new life to archives.
Who will jump on the opportunity? Probably those who are the most prone to invest in tech. I would guess Buzzfeed and the Jeff Bezos-owned Washington Post.
This post originally appeared at Monday Note.