As humans cede more and more control to algorithms, whether in the courtroom or on social media, the way they are built becomes increasingly important. The foundation of machine learning is data gathered by humans, and without careful consideration, the machines learn the same biases of their creators.
Sometimes bias is difficult to track, but other times it’s clear as the nose on someone’s face—like when it’s a face the algorithm is trying to process and judge. An online beauty contest called Beauty.ai, run by Youth Laboratories (that lists big names in tech like Nvidia and Microsoft as “partners and supporters” on the contest website), solicited 600,000 entries by saying they would be graded by artificial intelligence. The algorithm would look at wrinkles, face symmetry, amount of pimples and blemishes, race, and perceived age. However, race seemed to play a larger role than intended; of the 44 winners, 36 were white.
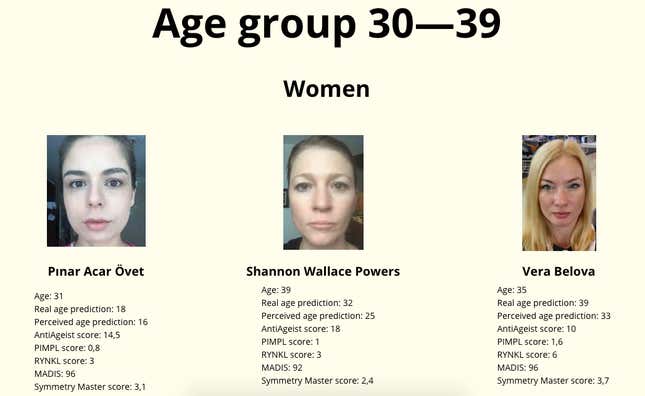
The tools used to judge the competition were powered by deep neural networks, a flavor of artificial intelligence that learns patterns from massive amounts of data. In this case, the algorithms would have been shown, for example, thousands or millions of photos with people who have wrinkles and people who don’t. The algorithm slowly learns similarities between different instances of wrinkles on faces, and can identify them in new photos. But if the algorithm learns primarily from pictures of white people, its accuracy drops when confronted with a darker face. (The same goes for the other judged traits, which each used a separate algorithm.)
While 75% of applicants were white and of European descent, according to Motherboard, that theoretically shouldn’t matter. To the machine, these aren’t people, but similar assortments of pixels. When pixels don’t follow the expected pattern, they could be dropped as a bad input or accidentally punished by the algorithm’s misjudgment. In other words, the beauty in the photos was being judged by an objective standard—but that objective standard was built from an aggregate of white people.
“It happens to be that color does matter in machine vision,” Alex Zhavoronkov, chief science officer of Beauty.ai, told Motherboard. “And for some population groups the data sets are lacking an adequate number of samples to be able to train the deep neural networks.”
The answer to this problem is better data. If the algorithms are shown a more diverse set of people, they’ll be better-equipped to recognize them later.
This same problem has been illustrated in Google’s DeepDream experiments. Google researchers programmed algorithms to process images of architecture, landscapes, and famous art to amplify whatever patterns they found. The results were fractal hellscapes, punctuated by faces of dogs. It turned out the algorithm was trained on the open-source database ImageNet, which has thousands of dog photos, so the AI became easier really good at recognizing dog patterns in other instances.
It’s an issue that has plagued Google and HP, and still continues to be seen in large and small AI pursuits.
“If a system is trained on photos of people who are overwhelmingly white, it will have a harder time recognizing non-white faces,” writes Kate Crawford, principal researcher at Microsoft Research New York City, in a New York Times op-ed. “So inclusivity matters—from who designs it to who sits on the company boards and which ethical perspectives are included. Otherwise, we risk constructing machine intelligence that mirrors a narrow and privileged vision of society, with its old, familiar biases and stereotypes.”
Beauty.ai will hold another AI beauty contest in October, and though Zhavoronkov says that better data needs to be made available to the public, it’s unclear whether the next contest will use a different data set.