Implicit biases are a well-documented and pernicious feature of human languages. These associations, which we’re often not even aware of, can be relatively harmless: We associate flowers with positive words and insects with negative ones, for example. But they can also encode human prejudices, such as when we associate positive words with European American names and negative words with African American ones.
New research from computer scientists at Princeton suggests that computers learning human languages will also inevitably learn those human biases. In a draft paper, researchers describe how they used a common language-learning algorithm to infer associations between English words. The results demonstrated biases similar to those found in traditional psychology research and across a variety of topics. In fact, the authors were able to replicate every implicit bias study they tested using the computer model.
For example, a gender bias was identified in male names being more strongly associated with words such as “management” and “salary”. Female names were more strongly associated with words such as “home” and “family”.
The authors of the Princeton study tested what is known as a machine-learning algorithm. This is the same kind of computer program that powers Google’s search interface, Apple’s Siri, and many other kinds of software that interact with human language.
Machine-learning algorithms can only learn by example. In this particular case, the researchers taught the algorithm using nearly a trillion words of English-language text extracted from the internet. The algorithm was not explicitly seeking out any bias. Rather it simply derived understanding of the words from their proximity to one another. The associations the algorithm learned are, in some sense, the literal structure of the English language, at least as it is used online.
To further drive this point home, the authors compared the strength of associations between the names of different occupations (“doctor”, “teacher”, etc.) and words indicative of women. (“female”, “woman”, etc.) Astonishingly, that simple association predicts very accurately the number of women working in each of those professions. Chicken-and-egg argument aside, it’s remarkable how effectively an algorithm which knows nothing about jobs or work effectively reconstructed an important dimension of human social organization.
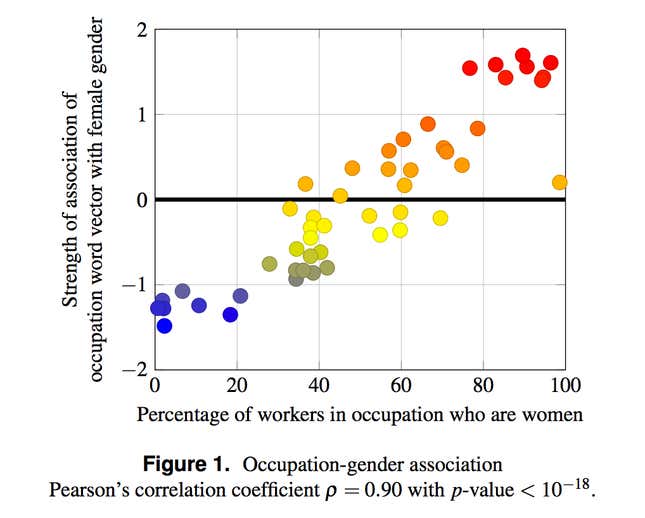
Machine-learning algorithms draw their power from their example-driven training process. Unfortunately, that process also means you can’t simply instruct the algorithms not to be biased. Unlike old-fashioned, human-programmed computer software, there is no switch one can push to say, “Don’t do this one thing.” In theory, it would be possible to train the algorithm with bias-free language samples, but even if it was possible to somehow create enough of those, they would, in some sense, be teaching the algorithm to misunderstand us.
Algorithms are increasingly central to decision-making in health care, criminal justice, advertising, and dozens of other fields. As these language-learning algorithms proliferate, it’s imperative that their designers are aware of the biases they encode into them. Earlier this year, ProPublica published a provocative story demonstrating racial bias in systems that assign “risk scores” to criminal defendants. Though these particular scores appear to have been based on more traditional statistical models, they illustrate how algorithmic biases can translate into a real-world harm. Biased risk scores could mean black people spend more time in jail than a similarly situated white person would have.
The solution to these problems is probably not to train algorithms to be speakers of a more ideal English language (or believers in a more ideal world), but rather in ensuring “algorithmic accountability” (pdf), which calls for layers of accountability for any decisions in which an algorithm is involved.
Evaluating outcomes independently of the algorithm, as ProPublica did in their analysis, can serve as a check on the biases of the machine. In the case that the results are truly biased, it may be necessary to override the results to compensate—a sort of “fake it until you make it” strategy for erasing the biases that creep into our algorithms.