A self-driving car is theoretically able to replace a human driver because it can see and react the way a human would—or better.
But AI researchers are now debating whether their software could be susceptible to “hacks” of real-world objects like stop signs, invisible to the human eye but seen by machines. In this scenario, a slight pattern or sticker applied to the sign would trick self-driving cars—or any AI—into misidentifying the sign as something else entirely, meaning the car would not necessarily slow or stop.
Researchers from Google, Pennsylvania State University, OpenAI, and elsewhere have been studying the theoretic application of these attacks, called “adversarial examples,” for years, and declared that they would be possible in the real world. By altering just 4% of an image, a Google paper showed that AI could be fooled into perceiving a different object 97% of the time. Now, an independently-published paper from the University of Illinois at Urbana Champaign has brought the discussion specifically to self-driving cars, but the conclusions of the paper are much less clear-cut.
Over a number of tests, the Illinois team printed fake stop signs with and without altered pixels and recorded videos approaching the signs as a self-driving car would. The resulting paper’s conclusion was that due to the different angles and sizes that the car would see the sign, a single pattern applied to a sign could not reliably fool a car.
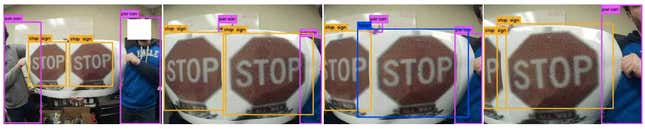
After looking at the research, other experts disagreed. Ian Goodfellow, a research scientist at Google who wrote much of the earliest work on adversarial examples, told Quartz that the paper’s results indicated that “the adversarial examples do clearly interfere” with the stop sign detector.
Goodfellow’s frequent and early co-author on adversarial example work, Nicolas Papernot from Pennsylvania State University, agreed with that assessment, adding that a more sophisticated attack would likely just try to apply a pattern that was crafted to work at different angles.
On Tuesday, in response to the paper, Elon Musk-backed AI research lab OpenAI did just that.
“We’ve created images that reliably fool neural network classifiers when viewed from varied scales and perspectives,” an OpenAI blog post read. “This challenges a claim from last week that self-driving cars would be hard to trick maliciously since they capture images from multiple scales, angles, perspectives, and the like.”
The research, whipped up in the five days since the Illinois paper was published, shows a printed picture of a kitten fooling image-recognition AI into thinking it’s a picture of a “monitor” or “desktop computer” from a number of angles, and as the picture gets closer and farther.
“When this paper claimed a simple fix, we were curious to reproduce the results for ourselves (and we did, in a sense). Then, we were curious if a determined attacker could break it, and we found that it was possible,” OpenAI researcher Anish Athalye told Quartz.
The rapid response to the Illinois paper illustrates two ideas: 1) never say never in AI research, 2) there will be a bumpy road ahead for autonomous vehicles. If fellow researchers can retaliate in a matter of days, autonomous automakers will find themselves locked in a daily game of cat-and-mouse against a slew of decentralized and constantly-evolving attacks in the real world.
Automakers might also have much simpler problems to fix before they can tackle adversarial examples. It’s entirely possible that a black marker and some poster board might be just as effective as a maliciously-crafted machine-learning attack—a Carnegie Mellon professor has documented how his Tesla mistook a highway junction sign as a 105 mile-per-hour speed limit.
David Forsyth, the Illinois professor who co-authored the paper, explained that what he worked on examined a small question—if traditional straightforward adversarial examples would work on self-driving cars— but was just the beginning of determining how adversarial examples worked in the wild.
“The correct conclusion is ‘more research is needed,'” Forsyth said.