A patient goes into the emergency room for a broken toe, is given a series of standardized tests, has their data fed into an algorithm, and—though they haven’t mentioned feeling depressed or having any suicidal thoughts—the machine identifies them as at high risk of suicide in the next week. Medical professionals must now broach the subject of suicide with someone who hasn’t yet asked for help, and find some way of intervening.
This scenario, where an actionable diagnosis comes not from a doctor’s evaluation or family member’s concern, but an algorithm, is an imminent reality. Last year, data scientists at Vanderbilt University Medical Center in Nashville, Tennessee, created a machine-learning algorithm (paywall) that uses hospital-admissions data, including age, gender, zip code, medication, and diagnostic history, to predict the likelihood of any given individual taking their own life. In trials using data gathered from more than 5,000 patients who had been admitted to the hospital for either self-harm or suicide attempts, the algorithm was 84% accurate at predicting whether someone would attempt suicide in the next week, and 80% accurate at predicting whether someone would attempt suicide within the next two years.
Colin Walsh, the Vanderbilt data scientist who led the project, wants to see this algorithm put to widespread use. Currently, most people in the US are only assessed for suicide risk when they actively seek psychiatric help, or exhibit clear-cut symptoms such as self-harm. Walsh hopes his algorithm will one day be applied in all medical settings, in order to catch depressive behavior early on, and give doctors the ability to proactively offer care to those who haven’t yet asked for it or shown obvious symptoms.
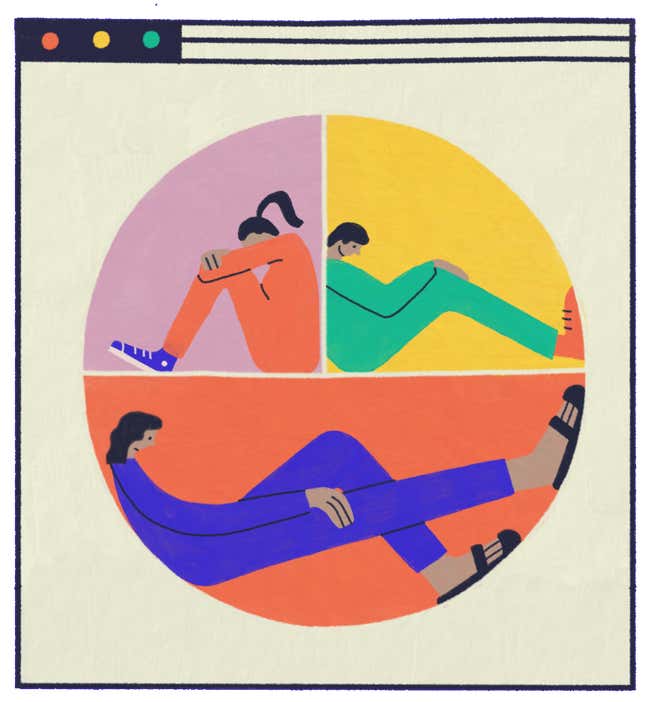
He’s not the only one interested in algorithms’ ability to predict suicide risk. Facebook has also developed pattern-recognition algorithms that will monitor users’ posts for risk of suicide or harm, and connect them with mental health services if necessary. The two projects rely on different data: Walsh has access to actual medical records, versus Facebook’s reliance on social media activity. Walsh’s project is also crucially different in that, by integrating with the US medical system, it opens up far more possibilities for how to respond to risk. At most, Facebook can put people in touch with mental health services, whereas doctors already have patients within their care and can offer an array of interventions and treatment programs.
Walsh is currently working with doctors to develop such an intervention program based on this algorithm. If the AI deems a patient to be at high risk of suicide, they might be asked to spend several days in a hospital under supervision. In certain cases, a doctor might deem hospitalization necessary regardless of whether or not the patient volunteers to be admitted. In lower-risk cases, the patient would be told of their analysis, and given information about available therapists and treatment plans. They would be offered an appointment with a psychiatrist and, a day or so after they’ve been discharged, a member of the medical facility would give them a call to check on their mental health.
This binary division of risk levels is imperfect, the Vanderbilt team acknowledges. For example, there are patients at intermediate risk, who are not clearly in immediate crisis, but are at considerable risk of suicide within a few weeks or months.
“We know we won’t get it right the first time,” says Walsh. “We’re trying to be transparent about that.” Nevertheless, he expects the program will be ready to roll out for clinical use in hospitals within the next two years.
Walsh consulted Warren Taylor, a psychiatrist and professor at Vanderbilt Psychiatric Hospital, on how the algorithm could work in practice, and he says the broken-toe scenario is conceivable. It’s fairly standard for someone to come in for a physical injury and leave with a diagnosis and prescription for extensive treatment for something else, Taylor says, often a previously undiagnosed addiction.
Most doctors are accustomed to keeping an eye out for the sorts of undiagnosed mental health conditions commonly seen in their fields, and psychiatrists are taught to assess suicide risk even among patients who don’t voluntarily mention suicidal thoughts. When they see patients at scheduled appointments, they consider factors such as demographics, medical history, and access to guns. Nevertheless, even psychiatrists are little better than a coin toss at predicting suicide attempts. And, Taylor notes, psychiatrists only have limited contact with many of the patients who are at risk of suicide; historically, he says, many of those who do attempt suicide often saw a doctor in the previous month, but comparatively few saw a psychiatrist.
“At the end of the day, we’re only human and our predictive powers are poor,” says Taylor. As such, the algorithm has the potential to make a drastic difference. The fact that so many suicidal patients see a doctor, if not a psychiatrist, during the critical period of their lives, means that the algorithm could be used among those many patients come into contact with the medical system but currently aren’t assessed for risk of suicide. If an AI with an 80% prediction success rate is used to assess risk of suicide every time a patient comes into contact with medical care, then, in theory, we would be able to predict, treat, and hopefully prevent far more suicide attempts.
Doctors aren’t used to relying on machines to make such delicate decisions, but Taylor is open to trying them out. “Anything that could help give us more information is something we should include in evaluation,” he says.
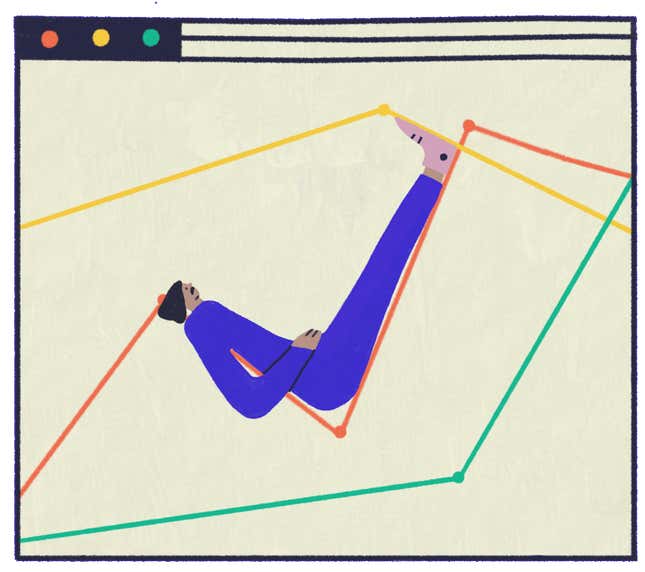
Though useful, the algorithm will likely raise ethical issues that cannot be foreseen or resolved until they play out in practice. “It’s not really outlined in our ethical or practice guidelines how to use AI yet,” McKernan says. For example, if, as Walsh hopes, all emergency room visitors are automatically run through the suicide-prediction algorithm, should they be told this is happening? McKernan says yes: all patients must be told that hospitals are monitoring data and assessing suicide risk, she adds. If a computer algorithm deems them to be at risk, they should know to expect a call.
As with all medical data, the question of who has access to the information raises another ethical minefield. In the case of the algorithm, Walsh says only doctors and those hospital staff treating the patient would know about the algorithm’s assessment; insurance companies and government bodies would not be privy to such knowledge.
It’s not yet obvious exactly what steps health care providers should take when a machine decides a patient is suicidal. But it’s clear that recognizing risk, and creating further interactions between patients and doctors, could have a huge impact in preventing suicide. McKernan says research she conducted on patients with chronic pain, done over a 20-year period (1998 to 2017), found that those who have a history of self-harm and whom the algorithm found to be at higher risk of suicidal thoughts spent far less time with medical professionals over the decades than those at lower risk of suicide. The results, which are due to be published in Arthritis Care & Research, show correlation rather than causation—after all, it may be that people at lower risk of suicide were more willing to engage with doctors—but nevertheless highlights a factor for doctors to analyze and consider. “It highlighted the importance of being in contact with [health care] providers,” McKernan says, and can “help direct what interventions we want to design,” like the programs Walsh is currently refining.
Though algorithms will undoubtedly inform and benefit patient care, McKernan is adamant that humans must hold ultimate responsibility for the overall assessment and treatment of a patient. The decision to keep a person under forced health care, for their own protection but potentially against their will, is extremely ethically sensitive. “When you hospitalize someone under those circumstances, you remove personal autonomy,” she says. A decision to override a patient’s claim that they’re not at risk, whether made by doctor or machine, must only happen under special and rare circumstances. “I’m not comfortable with the idea that an algorithm could override clinical judgment,” McKernan says.
Although the data behind every individual decision is based on too complex an analysis to identify any single factor for suicide risk, the algorithm can point to broad overall trends. For example, Walsh noticed a correlation between risk of suicide and melatonin prescriptions. While it’s unlikely that melatonin causes suicidal thoughts, the prescription is likely an indication of trouble sleeping, which itself is a key factor in suicidal risk.
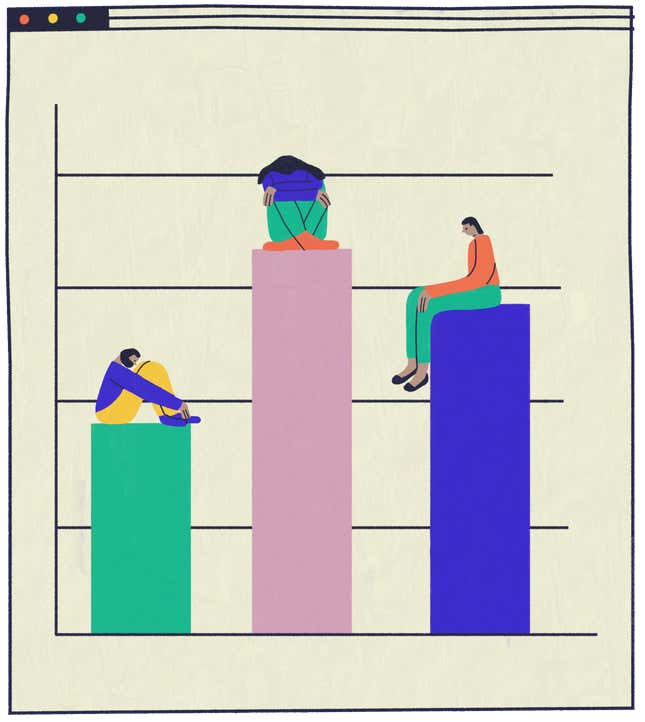
Ultimately, use of algorithms in a medical setting should be focused on developing more informed, data-driven, health care practices, rather than instituting hierarchical decision-making. “All models have times when they’re wrong,” says Walsh. “We need to learn from those examples as well.” Any prediction model should improve with time. If a physician overrides an algorithm’s decision and decides a patient is not at high suicide risk, that information can be fed back into the program to make it more accurate in the future. If the patient does later die by suicide, that should also be relayed to both algorithm and physician, with the hope that both will continue to improve their prediction accuracy.
The point is to inform a physician’s judgment, and potentially help doctors reconsider how they evaluate risk. “As humans, we get blinded by our own assumptions,” says Taylor. “People who are very depressed can be very good actors.” Someone who’s at risk of suicide can be attractive, successful, and smiling. Machines, unlike humans, aren’t misled by these distracting details and, thanks to their unique ability to focus on data rather than human traits, have an an uncanny ability to analyze and predict human behavior. Simply by virtue of thinking like a machine rather than a human, the algorithms can know us far better than we do ourselves.
Update (Sept. 6, 2018): This article has been updated to note that the study led by Lindsey McKernan is now peer-reviewed and due to be published.